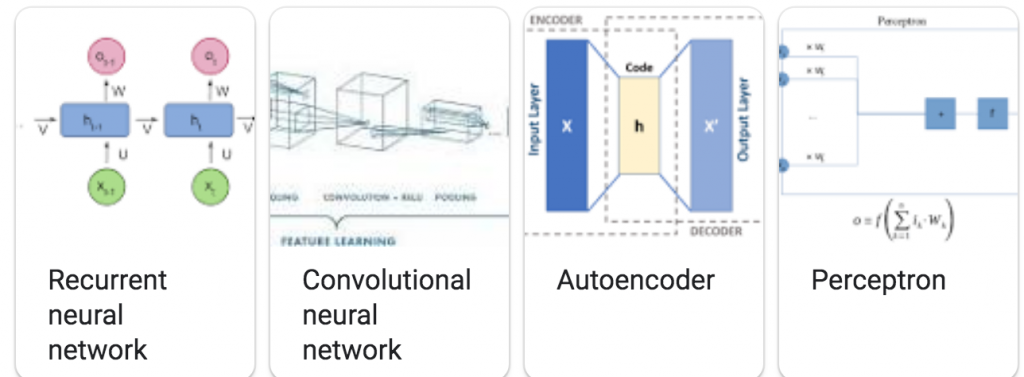
Types of deep learning algorithms. (Source: Forbes.com)
Forbes Columnist: How AI Will Learn from the Classroom of the World
Seeflection.com recently covered how Congress is considering training ArmyBots, but AI being able to teach itself whatever it wants without any oversight appears to be another alarming development. Rob Toews, an AI columnist at forbes.com, wrote a two-part article on the next generation of AI, which will digest data without human involvement.
The field of AI moves fast. It has only been eight years since the modern era of deep learning began at the 2012 ImageNet competition. The “breakneck pace” of development will increase and Toews writes that in five years, “the field of AI will look very different than it does today.”
This article highlights three emerging areas within AI that are poised to redefine the field—and society—in the years ahead. Here are some excerpts:
1. Unsupervised Learning
The dominant paradigm in the world of AI today is supervised learning. In supervised learning, AI models learn from datasets that humans have curated and labeled according to predefined categories. (The term “supervised learning” comes from the fact that human “supervisors” prepare the data in advance.)
While supervised learning has driven remarkable progress in AI over the past decade, from autonomous vehicles to voice assistants, it has serious limitations.
The process of manually labeling thousands or millions of data points can be enormously expensive and cumbersome. The fact that humans must label data by hand before machine learning models can ingest it has become a major bottleneck in AI.
Many AI leaders see unsupervised learning as the next great frontier in artificial intelligence. In the words of AI legend Yann LeCun:
“The next AI revolution will not be supervised.” UC Berkeley professor Jitenda Malik put it even more colorfully: “Labels are the opium of the machine learning researcher.”
In unsupervised learning, the algorithm learns about some parts of the world based on exposure to other parts of the world. By observing the behavior of, patterns among, and relationships between entities—for example, words in a text or people in a video—the system formulates an understanding of its environment. Researchers call this, “predicting everything from everything else.”
2. Federated Learning
One of the overarching challenges of the digital era is data privacy. Because data is the lifeblood of modern artificial intelligence, data privacy issues play a significant (and often limiting) role in AI’s trajectory.
Privacy-preserving artificial intelligence—methods that enable AI models to learn from datasets without compromising their privacy—is thus becoming an increasingly important pursuit. Perhaps the most promising approach to privacy-preserving AI is federated learning.
The concept of federated learning was first formulated by researchers at Google in early 2017. Over the past year, interest in federated learning has exploded: more than 1,000 research papers on federated learning were published in the first six months of 2020, compared to just 180 in all 2018.
3. Transformers
We have entered a golden era for natural language processing.
OpenAI’s release of GPT-3, the most powerful language model ever built, captivated the technology world this summer. It has set a new standard in NLP: it can write impressive poetry, generate functioning code, compose thoughtful business memos, write articles about itself, and so much more.
GPT-3 is just the latest (and largest) in a string of similarly architected NLP models—Google’s BERT, OpenAI’s GPT-2, Facebook’s RoBERTa and others—that are redefining what is possible in NLP.
The key technology breakthrough underlying this revolution in language AI is the Transformer.
The link to the second part of the Toews article is below.
read more at forbes.com
Leave A Comment