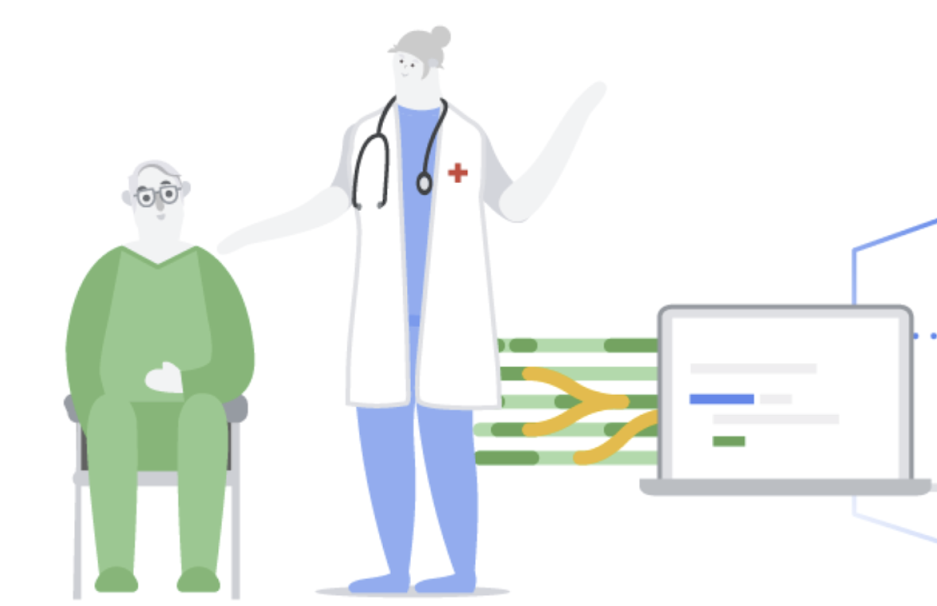
Using an AI system could lead to improved care, according to research. (Source: Google AI blog)
Medical AI System Can Prevent Prescription Errors, Predict Outcomes
Wikipedia describes Artificial intelligence (AI) in medicine as the use of complex algorithms and software to emulate human cognition in the analysis of complicated medical data. Specifically, AI is the ability of computer algorithms to approximate conclusions without direct human input. The positive impact of algorithms on the medical field has been nothing short of astounding.
According to an article by Ryan Daws for artificialintelligence-news.com, patients soon benefit from the use of AI to prevent dangerous prescription interactions. While statistically rare, prescriptions that are incorrect–or interact with other medications to cause severe reactions–can lead to emergency measures or even death.
A new AI system developed by researchers from Google and the University of California not only could help hospitals in preventing medical mishaps, but also in gauging how patients will respond to treatment.
In a blog post, Alvin Rajkomar MD, Research Scientist and Eyal Oren Ph.D., Product Manager, all of Google AI set out their work on using AI for medical “predictions.” The system is able to predict which conditions a patient is being treated for, based on a set of parameters.
“For example, if a doctor prescribed ceftriaxone and doxycycline for a patient with an elevated temperature, fever and cough, the model could identify these as signals that the patient was being treated for pneumonia,” the researchers wrote. “While no doctor, nurse, or pharmacist wants to make a mistake that harms a patient, research shows that 2% of hospitalized patients experience serious preventable medication-related incidents that can be life-threatening, cause permanent harm, or result in death. However, determining which medications are appropriate for any given patient at any given time is complex — doctors and pharmacists train for years before acquiring the skill.”
Predictive modeling using Electronic Healthcare Records (EHR) could prevent an array of problems that might not be anticipated by overworked physicians, especially considering that new medications are being introduced on a regular basis. According to the full paper published in the science journal Nature:
“It is widely suspected that use of such efforts, if successful, could provide major benefits not only for patient safety and quality but also in reducing healthcare costs,” the researchers wrote.
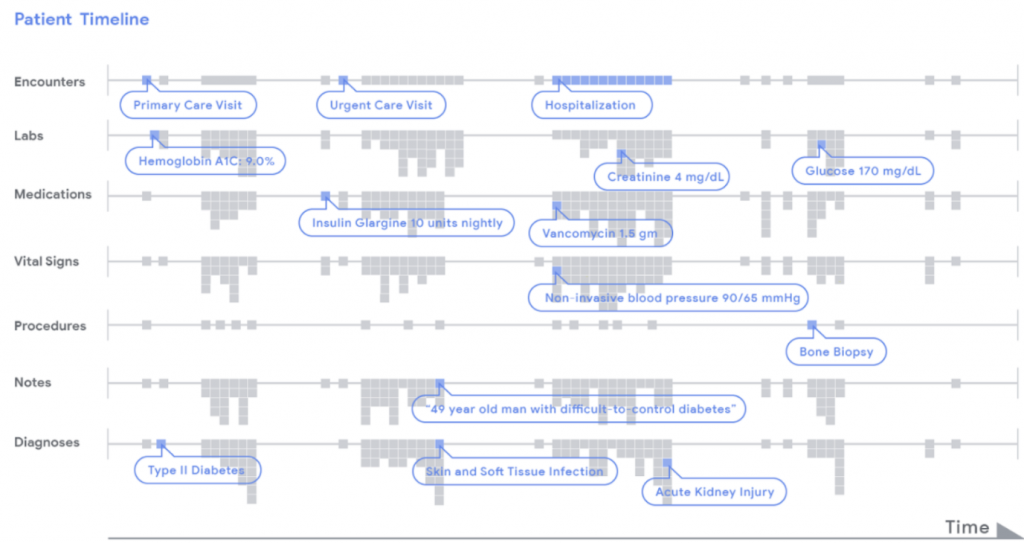
A diagram of a patient’s condition from admission to discharge shows the algorithm’s predictive accuracy. (Source: Google AI blog)
Current models are too simplistic to involve all of the data available, typically using anywhere from 5 to 27 data points, while the EHRs are chockfull of useful information. The group’s use of a deep-learning algorithm made it possible to consider thousands of pieces of data in the patients’ records.
“At the time of admission, an average admission had 137,882 tokens (discrete pieces of data that we define in the methods section), which increased markedly throughout the patient’s stay to 216,744 at discharge (Fig. 1),” the report said. “For predictions made at discharge, the information considered across both datasets included 46,864,534,945 tokens of EHR data.”
Using the data helped the model to make far more accurate predictions than other models, from the length of stay to readmissions to the likelihood of death.
“If a clinical team had to investigate patients predicted to be at high risk of dying, the rate of false alerts at each point in time was roughly halved by our model: at 24 h, the work-up-to-detection ratio of our model compared to the aEWS was 7.4 vs 14.3 (Hospital A) and 8.0 vs 15.4 (Hospital B). Moreover, the deep learning model achieved higher discrimination at every prediction time-point compared to the baseline models. The deep learning model attained a similar level of accuracy at 24–48 h earlier than the traditional models (Fig. 2).”
AI is expected to become a critical part of patient care in the future as these models become integrated into standard healthcare regimens.
read more at artificialintelligence-news.com
Leave A Comment