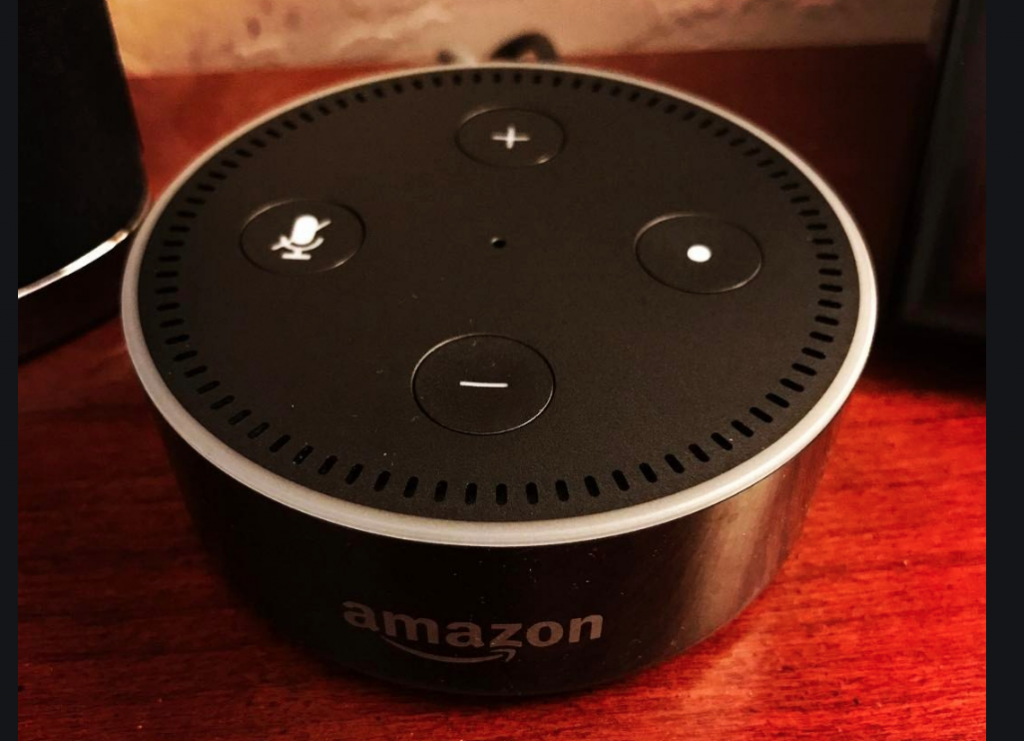
Voice-activated commands will be easier to follow for devices like Echo according to new research that enables better accuracy.
‘Beamforming’ Improves Devices’ Voice Recognition Accuracy in Tests
When your voice-driven assistant takes commands from you in the middle of a group of guests and there are multiple people speaking, how does it know which voice it needs to listen to? And how does it know which direction your voice is coming from?
In a technical paper scheduled to be presented next month at the International Conference on Acoustics, Speech, and Signal Processing (ICASSP), a group of Amazon researchers proposes an AI-driven approach to multiple-source localization or the problem of estimating a sound’s location using microphone audio. Kyle Wiggers from venturebeat.com had the inside story about the researchers’ findings in a recent story.
Addressing multiple-source localization is an indispensable step in developing sufficiently robust smart speakers, smart displays,and even video conferencing software. That’s because it’s at the core of beamforming, a technique that focuses a sound toward a microphone. Amazon’s own Echo lineup uses beamforming to improve voice recognition accuracy, as does Google’s Nest Hub and Apple’s HomePod.
Just as recording artists or producers have specific microphones for specific singers or vocal stylings, the same is true for the AI approach to listening. And any producer or recording engineer will tell you each voice and each microphone used for recording must be somewhat matched up for a quality product and outcome. Sound traveling toward an array of microphones will reach each microphone at a different time, which can be exploited to pinpoint the sources’ locations. With a single sound source, the computation is relatively straightforward, but with multiple sound sources, it becomes more complex. That’s where AI rides in to save the day according to the Amazon techs.
Various AI and machine learning solutions to the multiple-source localization problem have been proposed, but many have limitations.
When the number of possible sounds exceeds the number of model outputs, it’s difficult for the software to pick out which sound goes to which output.
“For example, if a model learns to associate a set of coordinates with one speaker and another set of coordinates with two other speakers, it’s unclear which output is associated with which speaker when the two other speakers talk at the same time.”
Using AI helps to direct which input/incoming sound should override another.
“The Amazon team’s model first localizes sounds to coarsely defined regions and then finely localizes them within those regions,” according to the VentureBeat.com story. “It considers a region active if it contains at least one source, and it assumes there can be at most one active source in any active region. Because each coarse region has a designated set of nodes in the model’s output layer, there can be no ambiguity about which sound source in a given region is associated with a location estimate.”
read more at venturebeat.com
Leave A Comment