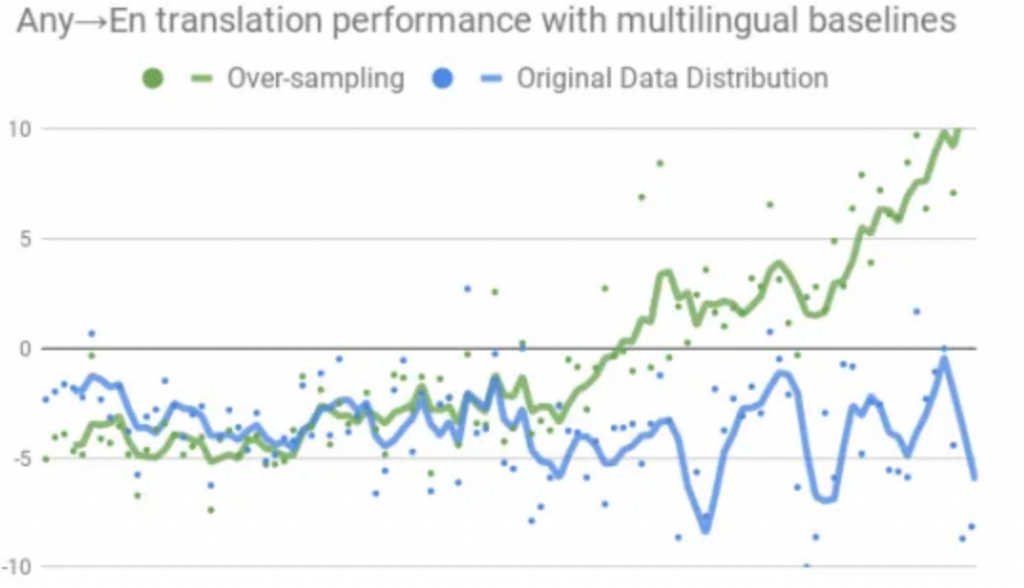
Figure 3: Effect of sampling strategy on the performance of multilingual models.
Facebook, Google Tout Multilingual Translation Technologies
Google has funded creation of a machine that will now translate 103 languages instantaneously, as reported in a story on syncedreview.com. Right now anyone can use Google Translate online for 100 of those languages, one to another at a time.
Graham Neubig, an Assistant Professor at the Language Technologies Institute of Carnegie Mellon University who works on natural language processing specifically multi-lingual models and natural language interfaces, explained his paper on the universal neural machine translation (NMT) system, created with Google.
Syncedreview.com started with this question:
How would you describe the universal neural machine translation (NMT) system?
Neubig: This paper does not propose a new technique for NMT per se. Rather it continues in a line of recent, and in my opinion exciting, work on building machine translation systems that can translate to or from multiple languages in the same model. Specifically, these models work by taking a neural machine translation system, and training it on data from many different languages at the same time. This line of work started out in 2015, and was further popularized by Google’s impressive paper in the space in 2017.
Recently, there has been a move towards “massively multilingual” systems that translate many languages at one time, including our paper training on 59 languages, and Google’s recent paper training on over 100 languages. The advantages of these systems are two-fold: (1) they can improve accuracy by learning from many different languages, and (2) they can reduce the computational footprint of deploying models by having only one model trained to translate many languages, as opposed to one model per language.
The paper Massively Multilingual Neural Machine Translation in the Wild: Findings and Challenges is on arXiv.
Facebook Says Pre-Trained Speech Recognition AI Can Translate as Well
Not to be outdone, Facebook also made news about training AI to translate languages, according to a story on venturebeat.com.
Multilingual masked language modeling involves training an AI model on text from several languages, and it’s a technique that’s been used to great effect. In March, a team introduced an architecture that can jointly learn sentence representations for 93 languages belonging to more than 30 different families. But most earlier work in language modeling has investigated cross-lingual transfer with a shared vocabulary across monolingual data sets. By contrast, Facebook researchers recently set out to explore whether linguistic knowledge transfer could be achieved with text from very different domains.
The work builds on Facebook’s extensive work in natural language processing, some of which it detailed in a blog post last month. The tech giant’s word2vec model uses raw audio to improve speech recognition, while its self-supervision model — ConvLM — recognizes words outside of its training lexicon with high accuracy. In a related development, Facebook recently demonstrated a machine learning system — Polyglot — that when given voice data is able to produce new speech samples in multiple languages, and researchers at the company devised ways to enhance Google’s BERT language model and achieve performance exceeding latest results across popular benchmark data sets.
The results will improve sharing information on financial transactions and medical information worldwide. Educational institutions can build stronger relationships when teachers can communicate with all the students assembled.
read more at syncedreview.com
Leave A Comment