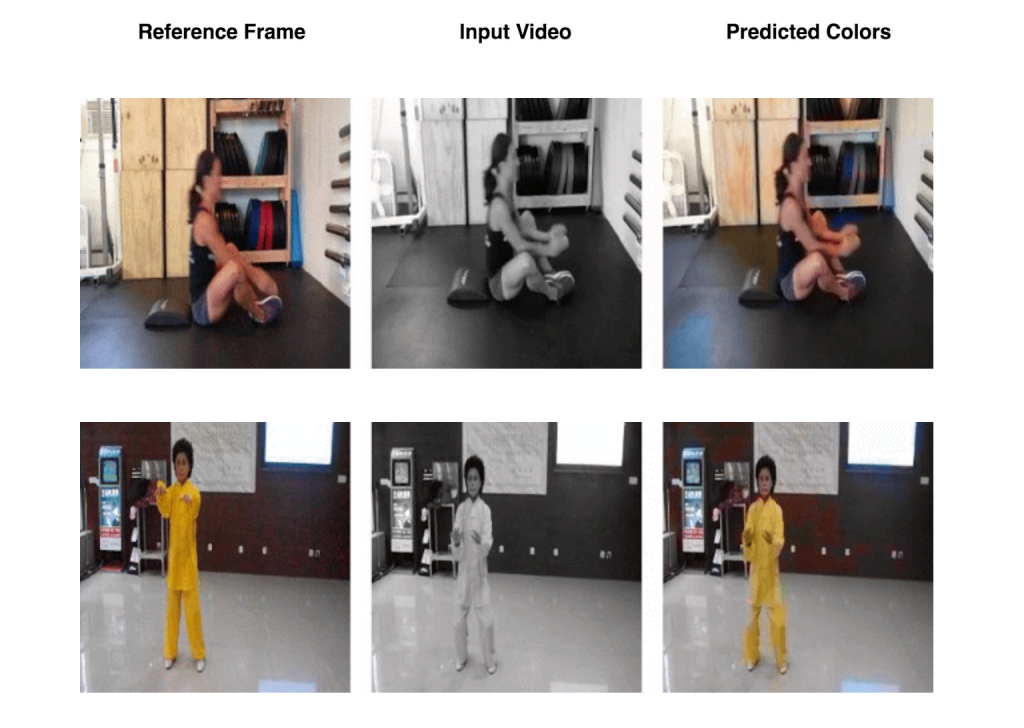
New Computer Vision Tracks Objects by IDing Color
A team of researchers at Google AI (formerly Google Research) have discovered how to reliably track objects in videos through colorizing footage, according to a blog post published June 27 summarizing the team’s paper Tracking Emerges by Colorizing Videos (pdf).
The AI not only learned to identify and track disparate objects,but also was able to successfully track the poses of human subjects, tracking wireframe “skeletons” throughout test videos. The team sees the advance as a basic step towards improving computer vision applications essential for recognizing activities, stylizing videos using AI, and improving robots’ abilities to interact with real-world objects.
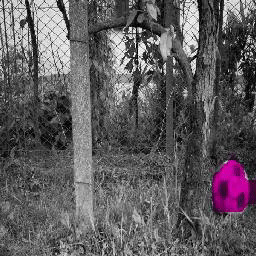
After marking an object to be tracked, the AI reliably follows it through the scene, even if occluded—such as the soccer ball passing behind trees. Via Google AI.
While computer vision AI has become adept at differentiating and recognizing objects in still images, tracking objects in video requires training neural networks on massive and laboriously detailed datasets, according to Google AI. Google’s AI, however, has taught itself how to track objects in video using a dataset of videos absent any of the annotation on objects or their positions needed for other methods.
Training the AI on the unlabeled Kinetics dataset featuring a variety of videos portraying everyday scenes, the Google AI team created a self-supervised convolutional neural network that was able to intuit and track objects in a scene through a simple means: color.
According to Google, the team hypothesized that color might be a useful means of training its AI to track objects, given the temporal coherency of color. In other words, unique objects and scenes captured in real-world video data tend to rarely change color (though “lights turning on suddenly” or other limited exceptions exist), meaning that color is a reliable metric for tracking an object throughout a video scene.
If the researchers could train the AI to learn the respective colors of objects within a given video and apply them throughout the clip, it would then be able, as the team surmised, to teach itself to track objects absent direct instruction using labelled data, or the “ground-truth” of the scene.
Rather than create an AI with the explicit goal of identifying and tracking objects, the team feeds the neural network desaturated videos from the dataset along with a single full-color still frame from the start of each video. The AI then learns to recolorize the full black-and-white video, inferring the colors of each frame using the sole full-color reference. With the goal of colorizing the video, the AI learns to track different components within a scene to ensure each is properly colored.
Above: From a single full-color reference frame (left), the AI can recolorize the remainder of a black-and-white video (right), developing an ‘understanding’ of object tracking in the process. Via Google AI.
After learning to colorize videos, the team’s trained AI was then able to consistently track moving objects or points specified as regions of interest.
In addition to tracking objects, Google AI’s trained neural network was also able to track human poses, analyzing footage of people from the JHMDB dataset to infer the position of a skeletal wireframe approximating pose.
Similarly to the colorization exercise the AI was trained on, the model can track changes in the human body throughout a video if only one initial frame from the video is tagged to show a subject’s joint skeleton (left).
According to Google AI’s post, “we found that the failures from our system are correlated with failures to colorize the video, which suggests that further improving the video colorization model can advance progress in self-supervised tracking.”
Advancements in computer vision AI able to parse out objects and identify poses have innumerable utilities and implications and, as with image recognition AI trained on still images, more accurate tracking in video AI in might be employed in uses ranging from autonomous vehicles to surveillance or assist in video editing.
Leave A Comment