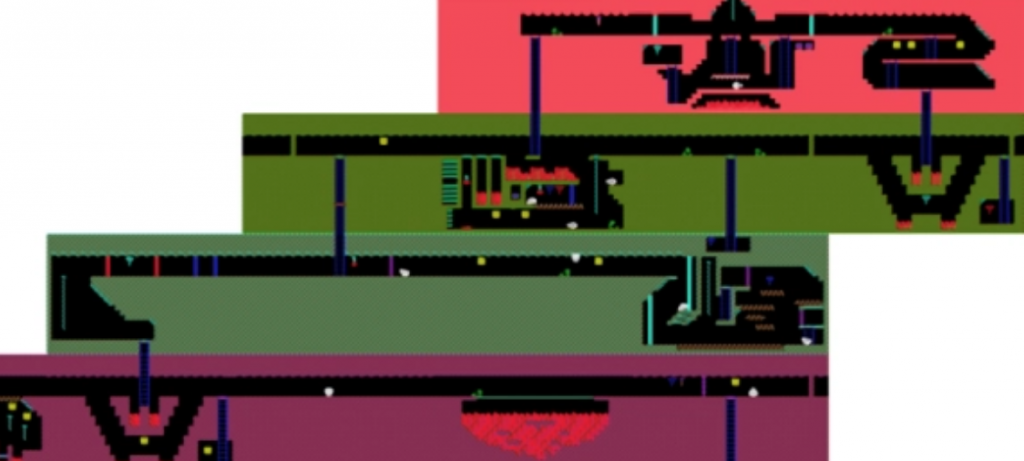
Map of level one on Montezuma’s Revenge. (Source: Wikimedia Foundation)
AI Researchers: Go-Explore Training Agents Figure out How to Beat Challenging Atari Games
It might be difficult to imagine how a program that can be trained on Atari games could move on to design better drugs for humans, but a story on venturebeat.com explains that it’s possible.
An article by Khari Johnson promotes the latest AI to beat video games and even text-based games.
In 2018, Uber AI Labs introduced Go-Explore, a family of algorithms that beat the Atari game Montezuma’s Revenge, a commonly accepted reinforcement learning challenge. Last year, Go-Explore was used to beat text-based games.
Now researchers from OpenAI and Uber AI Labs say Go-Explore has solved all previously unsolved games in the Atari 2600 benchmark from the Arcade Learning Environment, a collection of more than 50 games, including Pitfall and Pong. Go-Explore also quadruples the state-of-the-art score performance on Montezuma’s Revenge.
Training agents to navigate complex environments has been the main challenge for reinforcement learning. Machine learning milestones have included DeepMind’s AlphaGo and OpenAI’s Dota 2 beating human champions.
Researchers say recent Go-Explore advances could be applied to language models as well as drug design and training robots to navigate safely. In simulations, a robotic arm was able to successfully pick up an object and put it on one of four shelves, two of which are behind doors with latches. This demonstrates the “function of its overall design.”
“The insights presented in this work extend broadly; the simple decomposition of remembering previously found states, returning to them, and then exploring from them appears to be especially powerful, suggesting it may be a fundamental feature of learning in general. Harnessing these insights, either within or outside of the context of Go-Explore, may be essential to improve our ability to create generally intelligent agents,” reads a paper on the research published last week in Nature.
Detachment & Derailment
Researchers theorize that part of the problem is that agents in reinforcement learning environments forget how to get to places they have previously been (known as detachment) and generally fail to return to a state before exploring from it (known as derailment).
“To avoid detachment, Go-Explore builds an ‘archive’ of the different states it has visited in the environment, thus ensuring that states cannot be forgotten. Starting from an archive containing only the initial state, it builds this archive iteratively,” the paper reads. “By first returning before exploring, Go-Explore avoids derailment by minimizing exploration when returning (thus minimizing failure to return) after which it can focus purely on exploration.”
Last year Jeff Clune, who co-founded Uber AI Labs in 2017 before moving to OpenAI in 2020, said “catastrophic forgetting” is the central flaw of deep learning. Solving this problem could take researchers closer to artificial general intelligence (AGI).
read more at venturebeat.com
Leave A Comment