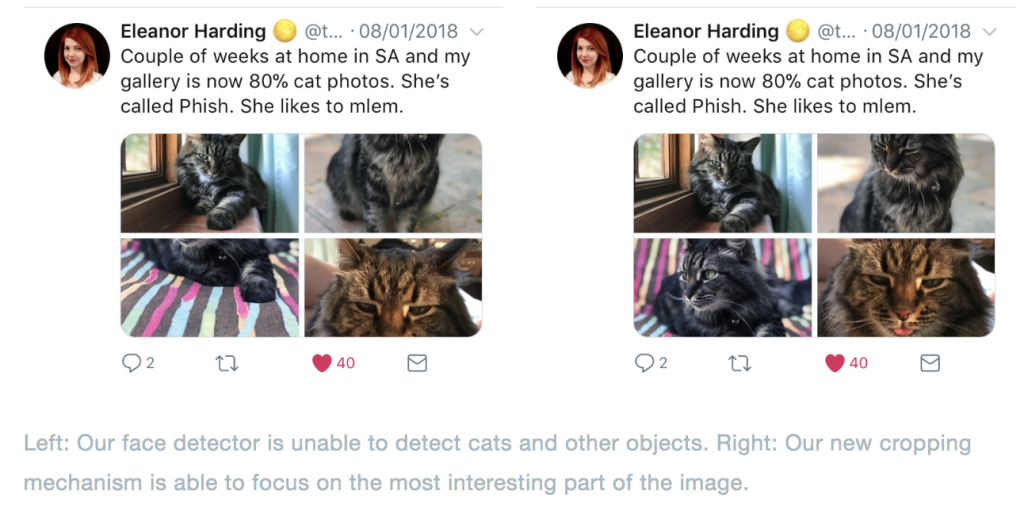
Users upset over algorithmic cropping of Twitter photos, as well as inaccuracies in posting images of people of color.
Twitter’s Photo Algorithm Favors White Posters over Those of Color
Seeflection has done several stories over the past two years about some of the drawbacks that plague algorithms. Sometimes they act more like machines instead of providing the human-level results sought. But there have also been instances of algorithms and their engineers being associated with built-in tendencies to discriminate against women and minorities. Now that question has been raised again by Khari Johnson in an article on venturebeat.com.
An algorithm Twitter uses to decide how photos are cropped in people’s timelines appears to be automatically electing to display the faces of white people over people with darker skin pigmentation. The apparent bias was discovered in recent days by Twitter users posting photos on the social media platform. A Twitter spokesperson said the company plans to reevaluate the algorithm and make the results available for others to review or replicate.
Twitter scrapped its face detection algorithm in 2017 for a saliency detection algorithm, which is made to predict the most important part of an image. A Twitter spokesperson said today that no race or gender bias was found in evaluation of the algorithm before it was deployed “but it’s clear we have more analysis to do.”
Back in 2018 two software engineers at Twitter wrote an article explaining how their algorithm decides how to crop a picture that will run on Twitter. Lucas Theis And Zuhan Wang wrote:
“The ability to share photos directly on Twitter has existed since 2011 and is now an integral part of the Twitter experience. Today, millions of images are uploaded to Twitter every day. However, they can come in all sorts of shapes and sizes, which presents a challenge for rendering a consistent UI experience. The photos in your timeline are cropped to improve consistency and to allow you to see more Tweets at a glance. How do we decide what to crop, that is, which part of the image do we show you?
“Previously, we used face detection to focus the view on the most prominent face we could find. While this is not an unreasonable heuristic, the approach has obvious limitations since not all images contain faces. Additionally, our face detector often missed faces and sometimes mistakenly detected faces when there were none. If no faces were found, we would focus the view on the center of the image. This could lead to awkwardly cropped preview images.
“First, we used a technique called knowledge distillation to train a smaller network to imitate the slower but more powerful network [3]. With this, an ensemble of large networks is used to generate predictions on a set of images. These predictions, together with some third-party saliency data, are then used to train a smaller, faster network.
Second, we developed a pruning technique to iteratively remove feature maps of the neural network which were costly to compute but did not contribute much to the performance. To decide which feature maps to prune, we computed the number of floating-point operations required for each feature map and combined it with an estimate of the performance loss that would be suffered by removing it. More details on our pruning approach can be found in our paper which has been released on arXiv [4].
They used a program that tracked the eyes of subjects as they watch the pixels on the screen, then engineers applied the data with the belief that it would work for all skin tones. It felt to some like they were using the algorithms to train us. We have included the link to this paper below.
On Saturday, algorithmic bias researcher Vinay Prabhu, whose recent work led MIT to scrap its 80 Million Tiny Images dataset, created a methodology for assessing the algorithm and was planning to share results via the recently created Twitter account Cropping Bias. However, following conversations with colleagues and hearing public reaction to the idea, Prabhu told VentureBeat he’s reconsidering whether to go forward with the assessment and questions the ethics of using saliency algorithms.
“Unbiased algorithmic saliency cropping is a pipe dream, and an ill-posed one at that. The very way in which the cropping problem is framed its fate is sealed, and there is no woke ‘unbiased’ algorithm implemented downstream that could fix it,” Prabhu said in a Medium post.
Prabhu isn’t sure his assessment would be beneficial, even if it was accurate.
“At the end of the day, if I do this extensive experimentation … what if it only serves to embolden apologists and people who are coming up with pseudo intellectual excuses and appropriating the 40:52 ratio as proof of the fact that it’s not racist? What if it further emboldens that argument? That would be exactly contrary to what I aspire to do. That’s my worst fear,” he said.
Johnson goes on to include in her article Twitter’s reaction to this lastest question about their algorithm’s intentions.
read more at venturebeat.com and on twitter.com’s blog
Leave A Comment