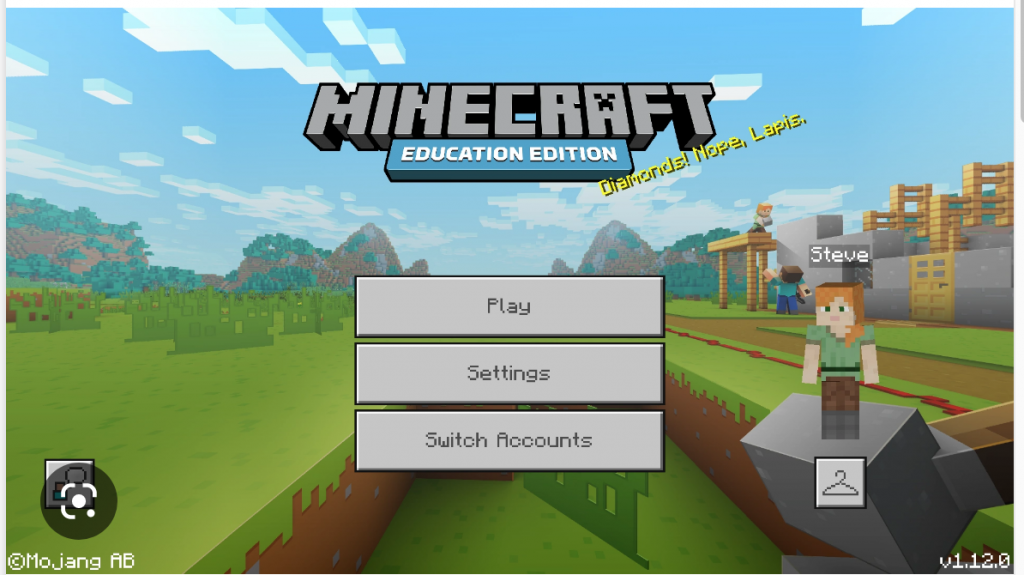
Researchers used 70,000 hours of videos to train this algorithm to dominate Minecraft.
OpenAI Builds the World’s Best Minecraft Player, Opens
Are you a Minecraft enthusiast? In the interest of full disclosure, I have never played this world-famous electronic video game. The idea of the game is to find materials in that video world and combine them to build objects. At least that’s how it is explained in a recent MIT Technology Review story.
OpenAI trained a neural network to play Minecraft, literally copying the human hand and finger movements on the game controller. The network reviewed 70,000 hours of video of people playing the popular computer game, leading to a powerful new technique that could be used to train machines to carry out a wide range of tasks by absorbing YouTube videos, which comprise a “vast and untapped source of training data.”
“The Minecraft AI learned to perform complicated sequences of keyboard and mouse clicks to complete tasks in the game, such as chopping down trees and crafting tools. It’s the first bot to craft so-called diamond tools, a task that typically takes good human players 20 minutes of high-speed clicking—or around 24,000 actions.
The result is a breakthrough for a technique known as imitation learning, in which neural networks are trained on how to perform tasks by watching humans do them. Imitation learning can be used to train AI to control robot arms, drive cars or navigate web pages.”
Scientists are hoping to replicate the success that GPT-3 had in upgrading its language processor.
“In the last few years we’ve seen the rise of this GPT-3 paradigm where we see amazing capabilities come from big models trained on enormous swathes of the internet,” says Bowen Baker at OpenAI, one of the team behind the new Minecraft bot. “A large part of that is because we’re modeling what humans do when they go online.”
Video Pre-Training Neural Networks
The author of this piece is Will Douglas Heaven and he explains the process called VPT, and what was required to get rolling. First, they got their people to come to play the game.
The team’s approach, called Video Pre-Training (VPT), speeds imitation learning by training another neural network to label videos automatically. They started by hiring crowdworkers to play Minecraft, then recorded their keyboard and mouse clicks alongside the video from their screens. This gave researchers 2,000 hours of annotated Minecraft play, which they used to train a model to match actions to the onscreen outcome. Clicking a mouse button in a certain situation makes the character swing its axe, for example.
The hope is this process can be duplicated for self-driving cars or even robots that shop for people. It’s an interesting piece and is loaded with optimistic results from a new approach to training AI. And that will also evolve into other more effective ways that AI can assist the world at large.
Not everyone is 100% on board with this process.
“This work is another testament to the power of scaling up models and training on massive datasets to get good performance,” says Natasha Jaques, who works on multi-agent reinforcement learning at Google and the University of California, Berkeley.
Large internet-sized data sets will certainly unlock new capabilities for AI, but Jaques is skeptical that data alone will solve problems that might crop up.
Baker and his colleagues think that collecting more than a million hours of Minecraft videos will make their AI even better. It’s probably the best Minecraft-playing bot yet, says Baker:
“But with more data and bigger models I would expect it to feel like you’re watching a human playing the game, as opposed to a baby AI trying to mimic a human.”
read more at technologyreview.com
Leave A Comment