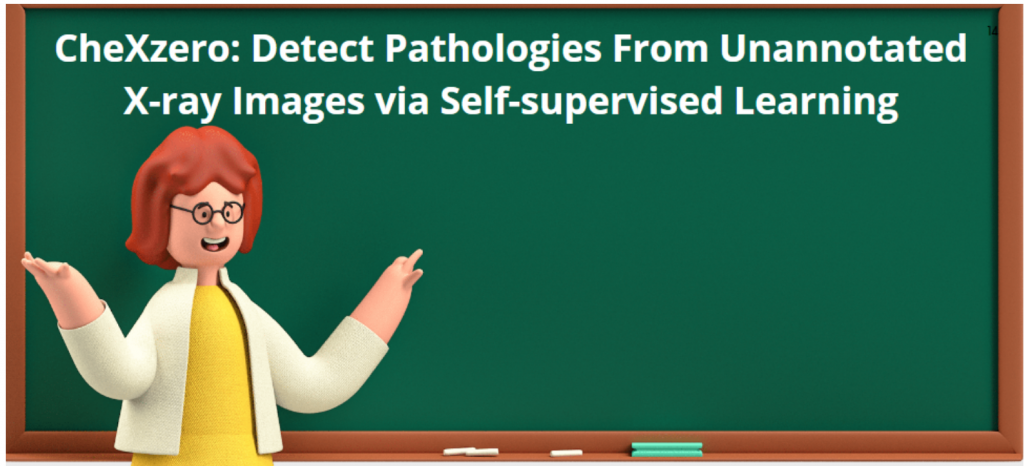
The new tool uses natural language descriptions from the accompanying clinical reports to identify diseases on chest X-rays.
AI Finds Diseases, Tumors, and Medical Abnormalities Previously Missed by Humans
In the past few years, we here at Seeflection.com have been nothing short of astounded at the rate at which AI and the medical professions have come together to create an entirely new universe regarding health and medical care. In some ways, AI has reinvented so many of the tools doctors have used to make their diagnoses to select the proper care for their patients.
The use of AI in training surgeons has been widely reported. The creation of new drug compounds that are now possible thanks to the addition of AI. Recently, we shared a piece about AI being able to read x-rays as well or better than human techs.
Now comes an article from scitechdaily.com that reports a newly developed diagnostic AI reads imaging far better than human radiologists when it comes to catching certain diseases just from the natural language reports that accompany the patient’s x-rays. AI is able to produce incredible results across almost all versions of medical imaging including cat scans, MRIs, etc.
Next Generation Arrives
Scientists from Harvard Medical School and Stanford University have created a diagnostic tool named CheXzero. According to a paper describing their work that was published in Nature Biomedical Engineering, researchers say it has performed on par with human radiologists in its ability to identify pathologies on chest X-rays. The group also made the model’s code openly accessible to other researchers.
To correctly detect pathologies during their “training,” the majority of AI algorithms need labeled datasets. Since this procedure requires extensive, often costly, and time-consuming annotation by human clinicians, it is particularly difficult for tasks involving the interpretation of medical images.
For instance, to label a chest X-ray dataset, expert radiologists had to look at hundreds of thousands of X-ray images one by one and explicitly annotate each one with the conditions detected. While more recent AI models have tried to address this labeling bottleneck by learning from unlabeled data in a “pre-training” stage, they eventually require fine-tuning on labeled data to achieve high performance.
You can already see the advantage in speed that AI and machine learning have over human technologists.
“We’re living in the early days of the next-generation medical AI models that are able to perform flexible tasks by directly learning from text,” said study lead investigator Pranav Rajpurkar, assistant professor of biomedical informatics in the Blavatnik Institute at HMS. “Up until now, most AI models have relied on manual annotation of huge amounts of data—to the tune of 100,000 images—to achieve high performance. Our method needs no such disease-specific annotations.
This use of machine learning/ AI technology has been shown it has the ability to help detect a wide variety of medical problems. Covid, cancer, Crohn’s disease, and many other diseases have already had advancements in early detection and treatment. Every day new maladies are being added to the list of medical issues being successfully addressed and in some cases, even cured through the use of this amazing technology.
read more at scitechdaily.com
Leave A Comment