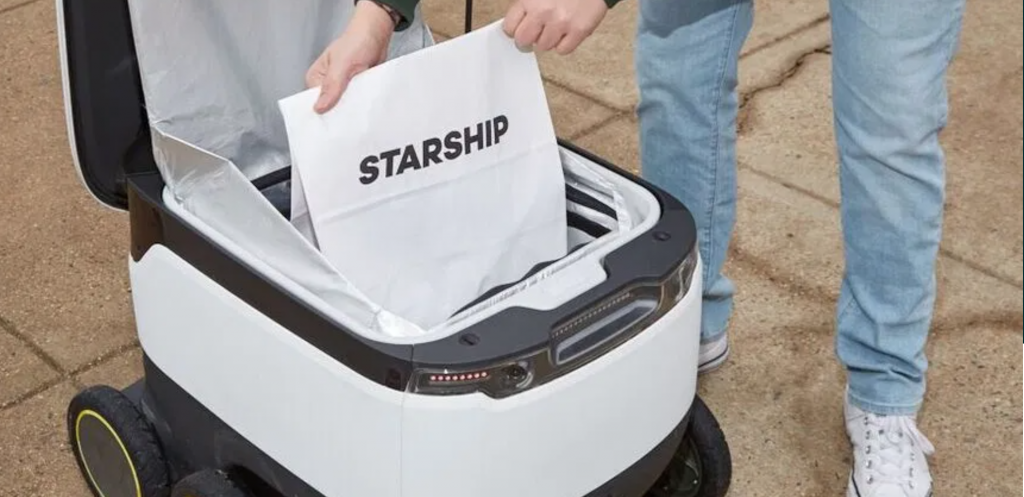
A Starship Technologies autonomous robot is just one example of AI-operated tech that addresses a problem during the COVID-19 pandemic. (Source: Starship Technologies)
Beyond Google’s Former Motto ‘Don’t Be Evil’: How Can AI Be ‘Good’?
What makes an AI project good? Is it the “goodness” of the domain of application, be it health, education, or environment? Is it the problem being solved (e.g. predicting natural disasters or detecting cancer earlier)? Is it the potential positive impact on society, and if so, how is that quantified? Or is it simply the good intentions of the person behind the project? The lack of a clear definition of AI for good opens the door to misunderstandings and misinterpretations, along with great chaos.
Of all the fantastic things AI has accomplished, it’s still far too early in the Tech Revolution to know exactly what to expect from AI’s evolution. We found an incredibly in-depth article from our friends at venturebeat.com that asks the question: What Makes AI For Good A Good Thing?
Artificial intelligence has taken a front seat during the global pandemic, spurring governments and private companies worldwide to propose AI solutions for everything from analyzing cough sounds to deploying disinfecting robots in hospitals. (You can find an article on AI and coughing in this week’s Seeflection.com coverage.)
These efforts are part of a wider trend that has been picking up momentum: the deployment of projects by companies, governments, universities and research institutes aiming to use AI for societal good. The goal of most of these programs is to deploy cutting-edge AI technologies to solve critical issues such as poverty, hunger, crime, and climate change, under the “AI for good” umbrella.
Sasha Luccioni’s article is loaded with information about how to think about what really makes up a “good AI.” Best practices in AI for good fall into two general categories — asking the right questions and including the right people. Generally speaking, here are some questions that need to be answered before developing an AI-for-good project:
Asking The Right Questions
Who will define the problem to be solved?
Is AI the right solution for the problem?
Where will the data come from?
What metrics will be used for measuring progress?
Who will use the solution?
Who will maintain the technology?
Who will make the ultimate decision based on the model’s predictions?
Who or what will be held accountable if the AI has unintended consequences?
While there is no guaranteed right answer to any of the questions above, they are a good sanity check before deploying such a complex and impactful technology as AI when vulnerable people and precarious situations are involved.
In promoting a project, companies need to be clear about its scope and limitations and not only focused on the potential benefits it can deliver. As with any AI project, they need to be transparent about the approach used, the reasoning behind this approach, and the advantages and disadvantages of the final model. External assessments should be carried out at different stages of the project to identify potential issues before they percolate through the project. These should cover aspects such as ethics and bias, but also potential human rights violations, and the feasibility of the proposed solution.
“A prime example is the now-infamous COMPAS (Correctional Offender Management Profiling for Alternative Sanctions) project, which various justice systems in the United States deployed,” the VentureBeat article pointed out. “The aim of the system was to help judges assess risk of inmate recidivism and to lighten the load on the overflowing incarceration system. Yet, the tool’s risk of recidivism score was calculated along with factors not necessarily tied to criminal behaviour, such as substance abuse and stability. After an in-depth ProPublica investigation of the tool in 2016 revealed the software’s undeniable bias against blacks, usage of the system was stonewalled.”
2. Including the right people
AI solutions are not deployed in a vacuum or in a research laboratory but involve real people who should be given a voice and ownership of the AI that is being deployed to “help’” them — and not just at the deployment phase of the project. In fact, it is vital to include non-governmental organizations (NGOs) and charities, since they have real-world knowledge of the problem at different levels and a clear idea of the solutions they require. They can also help deploy AI solutions so they have the biggest impact — populations trust organizations such as the Red Cross, sometimes more than local governments.
NGOs can also give precious feedback about how the AI is performing and propose improvements. This is essential, as AI-for-good solutions should include and empower local stakeholders who are close to the problem and to the populations affected by it. This should be done at all stages of the research and development process, from problem scoping to deployment. The two examples of successful AI-for-good initiatives I cited above (CompSusNet and Stats for Social Good) do just that, by including people from diverse, interdisciplinary backgrounds and engaging them in a meaningful way around impactful projects.
The article describes some AI for Good success stories and some AI that went off the rails. We have all had nightmares about AI run amuck. But many had dreams that produced AI advances that once seemed beyond possible in the real world, with more to come. And that’s all good.
read more at venturebeat.com
Leave A Comment