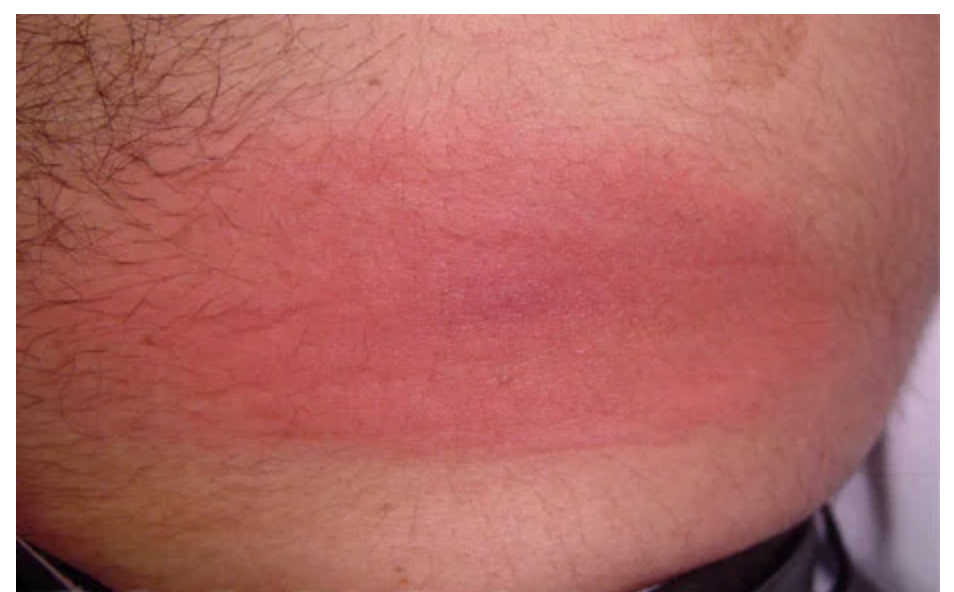
AI with deep learning examines erythema migrans from mobile phone photos.
New Study Shows Improved Lyme Detection Rates Using AI Photo Scans
If you are a resident of the Midwest or the eastern part of the U.S. then you are probably pretty familiar with the deer tick that causes Lyme disease.
The skin irritation can be confused with other similar rash signs and even doctors can misdiagnose them. The highly respected Johns Hopkins University posted an article about new ways that AI and deep learning programs are helping doctors detect erythema migrans (EM) or the red spots from Lyme disease.
It could help avoid the serious potential neurologic, rheumatologic, and cardiac complications of advanced disease.
Scientists developed and tested several deep learning computer models to accurately pick out EM from other dermatological conditions and normal skin. They “trained” the deep learning models to identify EM using images of non-EM rashes and normal skin available in the public domain and clinical photos of patients with EM.
Here is the kicker about this project: researchers trained the models to detect the disease from mobile phone images. Yes, you can text a selfie of your skin rash to them and they can diagnose you from home.
In the northeast, the number of Lyme disease cases continues to climb. More than 300,000 new cases of Lyme disease are diagnosed annually in the United States and treatment is most effective if it is caught early. Blood tests can show false negatives for Lyme disease detection, so researchers are turning to AI.
To address the EM recognition problem, the researchers applied an AI and DL approach to analyzing mobile phone images of rashes from patients suspected of having Lyme disease.
Evaluating the public domain images, the computer-enhanced image analysis system had an accuracy ranging from 72%, when choosing between EM and other rashes, to 94% when picking out EM from normal skin. For clinical images from patients already diagnosed with the disease, the system could positively identify an EM rash 86% of the time.
“Being able to analyze the rash images using artificial intelligence and deep learning enables us to more accurately diagnose that a patient has Lyme disease, determine the stage of the illness, and establish a more appropriate treatment plan,” says John Aucott, director of the Johns Hopkins Lyme Disease Clinical Research Center.
The study appears in Computers in Biology and Medicine.
read more at futurity.org
Leave A Comment