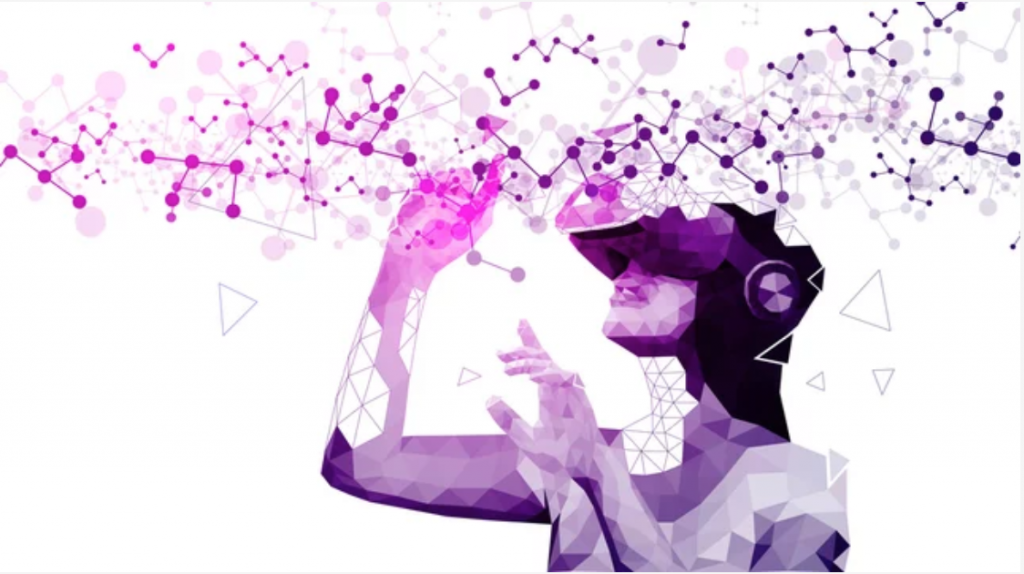
The Allen Institute for AI (AI2) Studies is adding more human-like senses to algorithms, which make them more intelligent. (Source: Adobe Stock)
‘Multimodality’ of Human Senses Assists in Training Algorithms
Making sense of life can be difficult when you are lacking one or more of the human senses. Sight, touch, sound, taste, smell, and the ability to express our thoughts are the tools that evolution gave us. Most of us have known or heard of many people who overcame the lack of any of their five senses to become quite successful at enjoying life. However, when you are an AI program you might be asked to become the savior of mankind without having all of the same tools that a human has. Without knowing enough, a robot is less likely to actually be able to save the world by not having experienced senses that humans possess.
Richard Yonck wrote a story for geekwire.com that addresses how we have managed to build algorithms that cannot experience the world the way humans do. The tools we use to train algorithms are not very similar to the people who are actually inventing them.
He points out the study found:
“The multimodality of combining image, sound, and other details greatly enhances our understanding of what’s happening, whether it’s on TV or in the real world.”
The same appears to be true for AI. A new question-answering model called MERLOT RESERVE enables out-of-the-box prediction, revealing strong commonsense understanding. It was recently developed by a team from the Allen Institute for Artificial Intelligence (AI2), the University of Washington, and the University of Edinburgh.
Part of a new generation of AI applications that enable semantic search, analysis, and question answering (QA), the system was trained by having it “watch” 20 million YouTube videos. The capabilities demonstrated are already being commercialized by startups such as Twelve Labs and Clipr.
It’s about Common Sense, Not Wine
Merlot Reserve ( RESERVE for short) stands for Multimodal Event Representation Learning Over Time, with Re-entrant Supervision of Events, and is built on the team’s previous MERLOT model. It was pre-trained on millions of videos, learning from the combined input of their images, audio, and transcriptions. Individual frames allow the system to learn spatially while video-level training gives it temporal information, training it about the relationships between elements that change over time.
Just as a restaurant’s chances are better whe the worker remember the admonition, “Location, Location, Location,” so it is for training AI: “Repetition, Repetition, Repetition.”
But the key is to improve how the program absorbs the repetition. One idea is sensors that can distinguish smells perhaps or a machine learning session with a hundred million hours of the various sounds the life on this planet makes. And then add in the ability to spot and identify most of those sounds with another program.
However, it’s not enough to simply load a bunch of facts and rules about how the world works into a system and expect it to work. The world is simply too complex. Humans, on the other hand, learn by interacting with our environment through our various senses from the moment we’re born. We incrementally build an understanding of what happens in the world and why. Some machine commonsense projects use a similar approach. For MERLOT and RESERVE, incorporating additional modalities provides extra information much as our senses do.
“The way AI processes things is going to be different from the way that humans do,” said computer scientist and project lead Rowan Zellers. “But there are some general principles that are going to be difficult to avoid if we want to build AI systems that are robust. I think multimodality is definitely in that bucket.”
Yonck goes on to explain in detail about RESERVE and he provides other information that is just too long to include here. The AI2 researchers say that the RESERVE project has proven to be more effective than its peers. And while it is a bit of a stiff read if one is not familiar with the terms, the study shows this kind of algorithm training concept will certainly be part of that teaching process from here forward.
The study’s paper will be presented at IEEE/CVF International Conference on Computer Vision and Pattern Recognition in June.
The authors of the project paper, “MERLOT RESERVE: Neural Script Knowledge through Vision and Language and Sound” are Rowan Zellers, Jiasen Lu, Ximing Lu, Youngjae Yu, Yanpeng Zhao, Mohammadreza Salehi, Aditya Kusupati, Jack Hessel, Ali Farhadi, and Yejin Choi. A demo for RESERVE can be found at AI2.
read more at geekwire.com
Leave A Comment