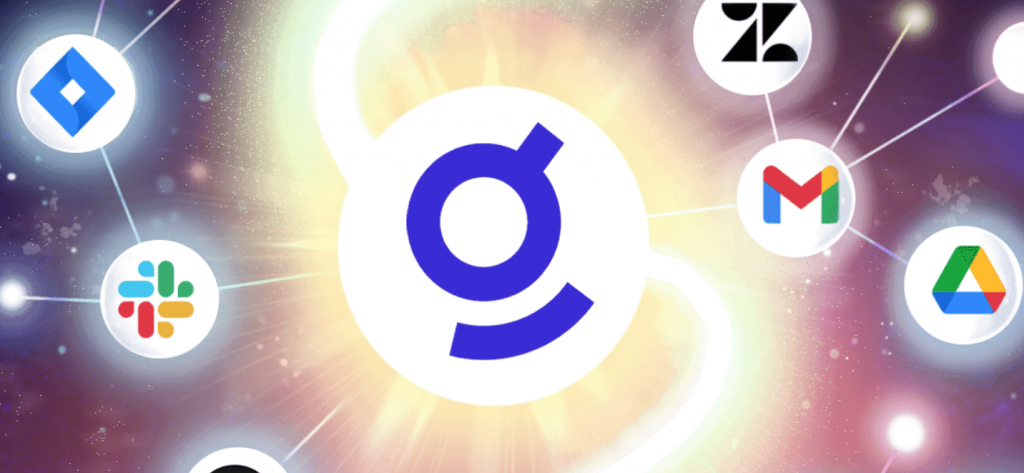
Start-ups like Glean, a ‘work assistant’ for companies, find AI training costs to be exorbitant. (Source: Glean.com)
Companies Create Innovations to Drive Down AI Training Costs
Gasoline, food costs, real estate, and even heating costs are going through the roof. But if you think you have had it rough battling the costs of living lately, wait till you hear how much it costs to use the best AI for your projects.
This week, Will Knight has a piece in wired.com on the rising costs of AI around the world. For instance:
CALVIN QI, who works at a search startup called Glean, would love to use the latest artificial intelligence algorithms to improve his company’s products. Glean provides tools for searching through applications like Gmail, Slack, and Salesforce. Qi says new AI techniques for parsing language would help Glean’s customers unearth the right file or conversation a lot faster.
Training an AI algorithm costs several million dollars, so Glean uses smaller, less capable AI models that can’t extract as much meaning from text. Imagine the amount of information that goes undiscovered due to the rising costs companies like Glean are missing out on.
Next Knight goes on to explain the best AI on the market which is called GPT-3. And it is costly.
Consider OpenAI’s language model GPT-3, a large, mathematically simulated neural network that was fed reams of text scraped from the web. GPT-3 can find statistical patterns that predict, with striking coherence, which words should follow others. Out of the box, GPT-3 is significantly better than previous AI models at tasks such as answering questions, summarizing text, and correcting grammatical errors. By one measure, it is 1,000 times more capable than its predecessor, GPT-2. But training GPT-3 costs, by some estimates, almost $5 million.
It’s hard to come up with that kind of financial support on an unproven project.
“If GPT-3 were accessible and cheap, it would totally supercharge our search engine,” Qi says. “That would be really, really powerful.”
Cloud Costs Are Sky High
Big companies have, of course, always had advantages in terms of budget, scale, and reach. Large amounts of computer power are critical in industries like drug discovery. Getting access to that much cloud power is costly.
Dan McCreary leads a team within one division of Optum, a health IT company, that uses language models to analyze transcripts of calls in order to identify higher-risk patients or recommend referrals. He says even training a language model that is one-thousandth the size of GPT-3 can quickly eat up the team’s budget. Models need to be trained for specific tasks and can cost more than $50,000, paid to cloud computing companies to rent their computers and programs.
To scale up further, Microsoft said this week it had built a language model more than twice as large as GPT-3 with the support of Nvidia. Researchers in China say they’ve built a language model that is four times larger than that. The Chinese rely on government investments. But when start-ups are relying on Series A investors to show up, it means the U.S. falls farther behind.
Dozens of companies are trying to innovate cost-saving techniques using specialized computer chips for both training and running AI programs. Qi of Glean and McCreary of Optum are both talking to Mosaic ML, a startup from MIT that is developing software tricks designed to increase the efficiency of machine-learning training.
After reading the details of so many of these financial problems that even multi-million dollar companies are dealing with, it makes $4-a-gallon gas look a little less problematic.
read more at wired.com
Leave A Comment