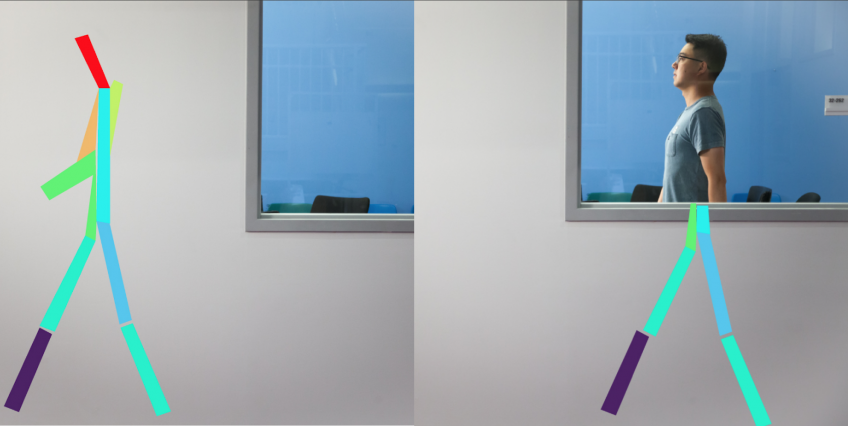
MIT Uses WiFi with AI to Detect Objects
In recent MIT project, researchers demonstrated the ability to see and even identify people through walls using radio waves similar to the WiFi signals that pervade the modern landscape.
According to a release by MIT News, the MIT Computer Science and Artificial Intelligence Laboratory (CSAIL) used RF waves in combination with traditional video footage to train a neural network to see people in a room, detecting the movements and posture of each including those partially or entirely occluded by walls and other barriers. Detailed in a paper due to be presented at the Conference on Computer Vision and Pattern Recognition (CVPR) this week in Salt Lake City, the team hopes that the technology might be further developed and used in healthcare applications.
Led by CSAIL’s Professor Dina Katabi, the research team developed its RF-Pose AI through a novel system that yielded results so precise that the system could not only detect people and their movements at rates approaching visual cameras but even identify the study’s volunteers. While most neural networks now rely on meticulously hand-labeled datasets to feed neural networks with only the most relevant data, RF data is far more difficult for human researchers to integrate visually. While a computer vision neural network could be trained to identify giraffes by, say, a set of images of giraffes appropriately labeled as such, labeling RF data poses the unique challenge of being nearly incomprehensible to human eyes.
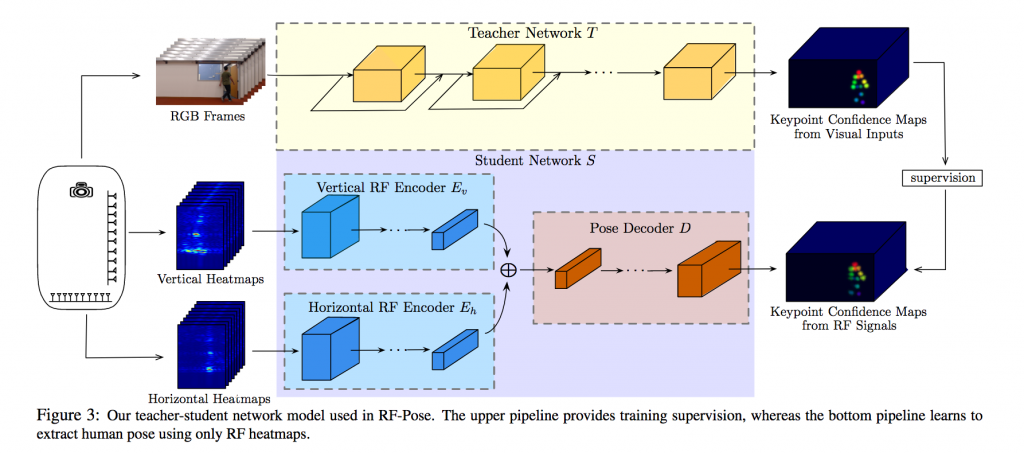
Above: A graphic from the paper outlining the structure of the team’s neural network. Via MIT.
To train the RF-Pose AI, researchers placed human volunteers throughout an indoor environment, recording both visual images with a webcam and simultaneously recording the RF readouts from an array of radio sensors. By pairing the easily categorized visual images with the radio data, the researchers had thousands of points of data and were able to create a neural network that could train itself to interpret a human’s position and movement in a given RF dataset. RF-Pose analyzes radio data to generate a 2D “stick figure” display of people within a room.
To the surprise of researchers, the RF-Pose AI was capable not only of tracking people in a scene with radio alone near the accuracy of the video-paired data, but could even see through walls and other obstacles. The AI had taught itself so efficiently to identify features of the radio data that in some cases it could exceed the capabilities of the training set, given that video input would obviously be incapable of identifying people behind a wall. “If you think of the computer vision system as the teacher, this is a truly fascinating example of the student outperforming the teacher,” said MIT Professor Antonio Torralba who participated in the study.
In addition to locating people and analyzing their movements, RF-Pose can also reliably identify individuals despite its seemingly crude 2D readout. In addition to more commonly known ways of identifying people such as fingerprints, iris scans, or facial recognition, an individual’s gait and other physical characteristics can also be highly unique biometric traits. From a set of 100 volunteers RF-Pose trained on, the AI could positively identify individuals 84.4% of the time with no meaningful effect on the system’s accuracy if the people were hidden behind walls.
While the security implications of RF-Pose are startling given that criminal or state actors could use similar AI in new surveillance systems or might even find ways of leveraging the world’s bounty of existing WiFi antennae to snoop on unsuspecting targets, the team seeks to use the tech for healthcare.
According to MIT News, the team hopes that the technology can be used to detect and treat movement-related diseases, such as multiple sclerosis, Parkinson’s and muscular dystrophy, by automatically tracking and analyzing patients’ movements in a hospital or at home. Similarly, the technology might be a valuable asset for caregivers of ill or elderly people, as the technology could help monitor people at risk of falls, epilepsy or other conditions.
“We’ve seen that monitoring patients’ walking speed and ability to do basic activities on their own gives health care providers a window into their lives that they didn’t have before, which could be meaningful for a whole range of diseases,” Katabi told MIT News. “A key advantage of our approach is that patients do not have to wear sensors or remember to charge their devices.” PhD candidate Hang Zhao concurred, adding that “by using this combination of visual data and AI to see through walls, we can enable better scene understanding and smarter environments to live safer, more productive lives.”
The CSAIL team seeks to continue to improve RF-Pose, eventually honing the network or similar projects to a degree enabling full 3D representations of people and with enough accuracy to spot and diagnose small disease-related movements such as tremors.
Leave A Comment