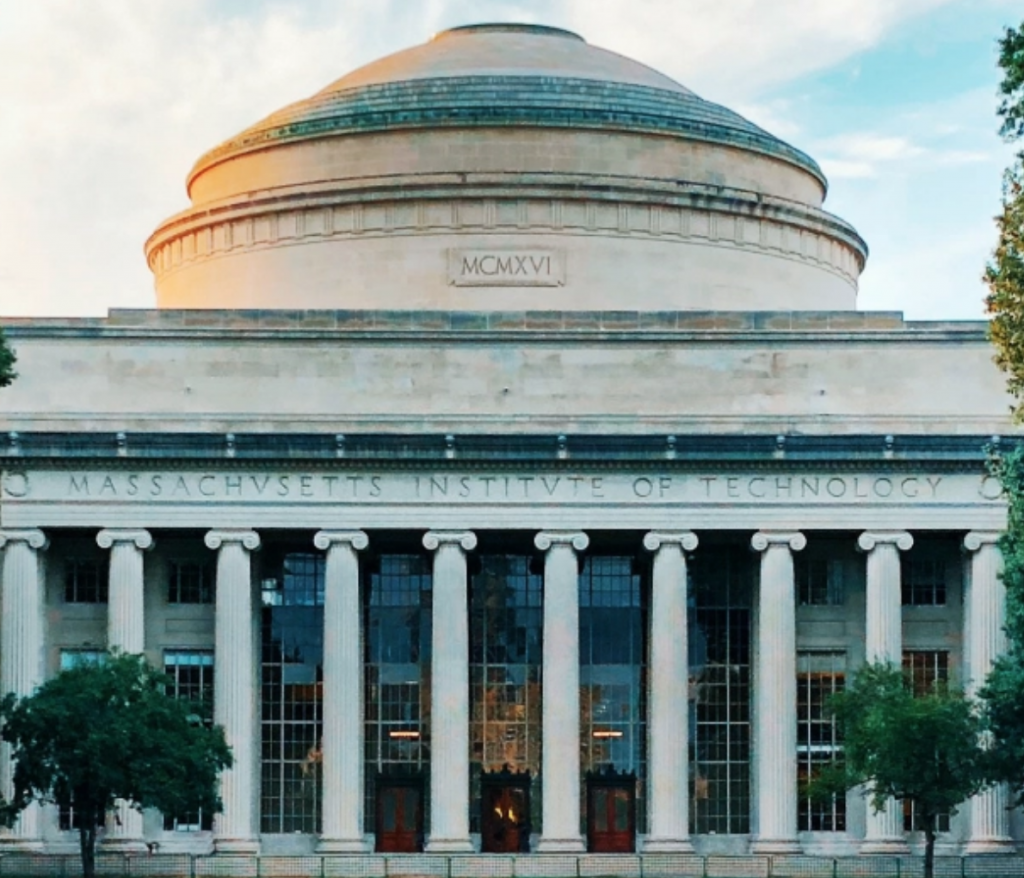
MIT is one of the well-financed learning centers for students.
Large Universities Gain Advantage, Creating ‘Compute Network’ Haves and Have Nots
The fact that wealthier universities and companies have an advantage in deep learning is not surprising. Large modern networks like AlphaGo Zero and GPT-3 can require millions of dollars in compute for training. And a December 2019 analysis labeled Google, Stanford University, MIT, Carnegie Mellon University, UC Berkeley,\ and Microsoft as the top six contributors at leading AI research conferences.
At the same time, smaller schools often lack the financial resources to consider deep learning applications. This limitation can define the kinds of AI that researchers in academia explore or accelerate brain drain to Big Tech companies with plenty of money to compete for top AI talent.
Couple that revelation from Khari Johnson’s article in venturebeat.com with the charges over the past decade indicating that racism is being programmed into algorithms, mostly on purpose, and it’s indisputable that universities have a deeper problem.
“Exploiting the sudden rise of deep learning due to an unanticipated usage of GPUs since 2012, we find that AI is increasingly being shaped by a few actors, and these actors are mostly affiliated with either large technology firms or elite universities,” their paper reads. “To truly ‘democratize’ AI, a concerted effort by policymakers, academic institutions, and firm-level actors is needed to tackle the compute divide.”
Just what we need in America. More inequality. But that is how our system works isn’t it? The rich get richer and the poor get less able to research and develop. You must have the financial clout to afford the same opportunities as the larger competitors you face.
Nur Ahmed and Muntasir Wahed summarized their findings and recommendations in a paper titled “The De-democratization of AI: Deep Learning and the Compute Divide in Artificial Intelligence Research.” The paper was published recently on arXiv and presented in late October at Strategic Management Society, a business research conference.
Confirming this opportunity gap, the paper found that universities ranked 301-500 by U.S. News and World Report have published on average six fewer papers at AI research conferences — or 25% fewer than a counterfactual estimator — since the rise of deep learning. Fortune 500 companies, Big Tech leaders, and elite universities saw dramatically different trends.
“To the best of our knowledge, this is the first study that finds evidence that an increased need for specialized equipment can result in ‘haves and have-nots’ in a scientific field,” the paper reads. “We contend that the rise of deep learning increases the importance of compute and data drastically, which, in turn, heightens the barriers of entry by increasing the costs of knowledge production.”
The only way forward for our centers of higher learning is to try and level the digital compute field. Johnson writes:
“When it comes to solutions, the coauthors say their findings present “concrete evidence” of the need for a national AI research cloud. In June, major universities, tech companies, and members of the U.S. Senate backed the idea of a national AI research cloud. Shared public datasets that can help train and test AI models will be particularly beneficial for resource-constrained organizations.”
The paper asserts that the U.S. government should help universities by extending shared public datasets and other resources. Groups like the Defense Innovation Board and National Security Commission on AI (NSCAI) have advised the Pentagon and Congress to increase public-private partnerships, government funding, and outreach to developers working remotely as a way to attract talent from nontraditional backgrounds.
Ahmed and Wahed’s paper also follows a survey of more than 200 computer science department chairs on the impact of industry on academia.
You can find Johnson’s information-packed article at the link below.
read more at venturebeat.com
Leave A Comment