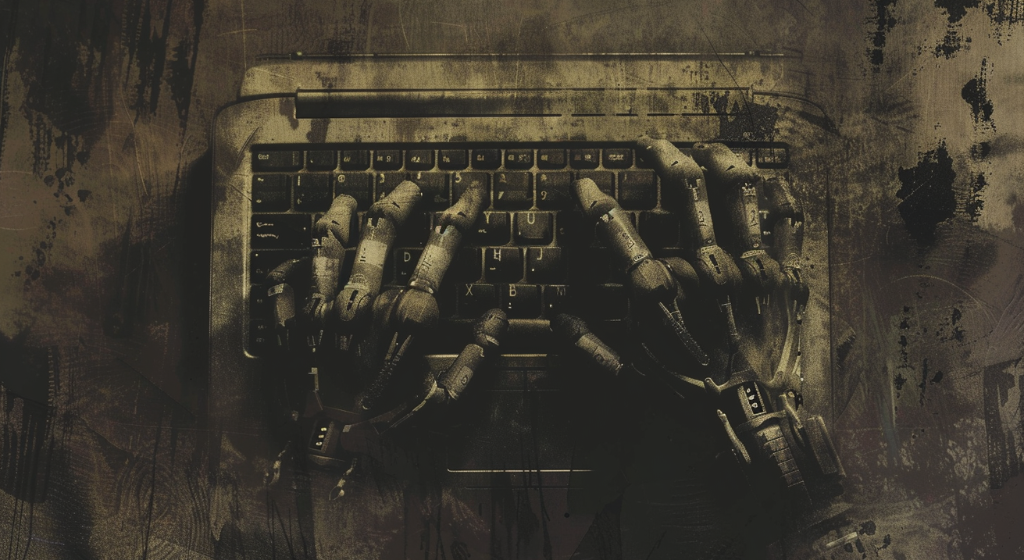
Detecting LLM use is crucial because these models often fabricate references, provide inaccurate summaries, and make false yet convincing claims, but as awareness of their telltale marker words grows, human editors will become more adept at removing these indicators from generated text before publication. (Source: Image by RR)
Groundbreaking Study Identifies Key Vocabulary Shifts in Academic Writing
Researchers from Germany’s University of Tübingen and Northwestern University have developed a novel method to estimate large language model (LLM) usage in scientific writing by identifying “excess words” that surged in frequency during the LLM era (2023-2024). Analyzing 14 million paper abstracts on PubMed, they discovered that words like “delves,” “showcasing,” and “underscores” significantly increased in usage, suggesting that at least 10 percent of 2024 abstracts were generated with LLM assistance. These findings, as reported in wired.com, indicate a marked change in vocabulary, primarily involving style words such as verbs, adjectives, and adverbs, rather than nouns associated with major world events like pandemics.
The study highlights that these vocabulary changes were unprecedented in both quality and quantity, pointing to the influence of LLMs on scientific writing. Unlike pre-LLM periods where sudden vocabulary shifts were tied to global health crises, the post-LLM era saw a widespread increase in certain style words without any common link to world events. The researchers used the pre-2023 abstracts as a control group to demonstrate the overall change in vocabulary choice in the post-LLM era.
By identifying hundreds of marker words that became more common in the post-LLM era, the researchers suggest that detecting LLM use in scientific writing can sometimes be straightforward. They estimate that LLM-assisted abstracts are prevalent, particularly in countries like China, South Korea, and Taiwan, where LLMs might aid non-native English speakers in editing their texts. In contrast, native English speakers may be better at removing these marker words, potentially masking their LLM usage.
The importance of detecting LLM use lies in the fact that LLMs are known for fabricating references, providing inaccurate summaries, and making false claims that sound authoritative. As awareness of LLM marker words grows, human editors might become more adept at eliminating these words from generated text. Future LLMs could even conduct frequency analysis to minimize marker words, making their outputs appear more human-like, which could necessitate more sophisticated methods for identifying AI-generated content.
read more at wired.com
Leave A Comment