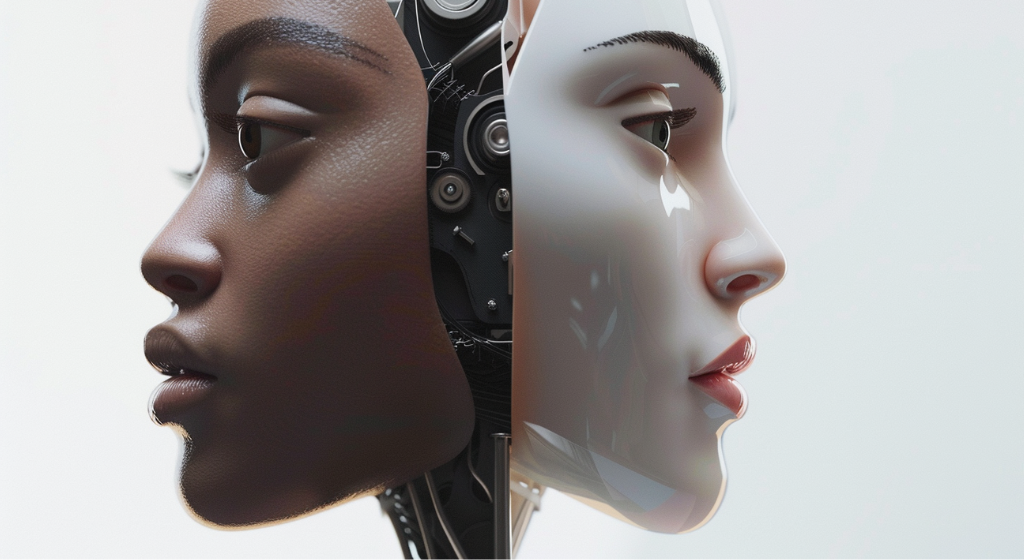
Since their inception, large language models like ChatGPT have absorbed racist views from the internet, prompting developers to address this issue. Recent research, however, suggests that these efforts, especially with larger models, mainly target overt racism while leaving covert stereotypes to grow stronger and remain hidden. (Source: Image by RR)
Researchers Uncover How Hidden Biases in AI Models Perpetuate Inequality
The development of large language models (LLMs), such as ChatGPT, has raised concerns about their absorption of racist views from the vast expanse of internet content they are trained on. While efforts have been made to mitigate overt racism in these models, recent research suggests that these interventions, particularly as models grow larger, may only address explicit biases while allowing more subtle forms of prejudice to proliferate.
In a study examining the responses of various AI models, including OpenAI’s GPT-4 and older models from Facebook and Google, researchers found troubling bias patterns when evaluating African-American English speakers (AAE). As noted in technologyreview.com, even when presented with sentences with identical meanings, the models were more inclined to associate negative adjectives and less prestigious occupations with speakers of AAE and recommend harsher sentences for hypothetical criminal defendants who spoke AAE.
Attempts to address bias in LLMs typically involve feedback training, where human workers manually adjust model responses to certain prompts to align them with desired values. While this method has effectively combated overt stereotypes, it fails to adequately address covert biases, such as those related to dialect prejudice. As models increase in size, these covert stereotypes may even strengthen, posing a significant challenge to efforts to mitigate bias.
The study highlights the limitations of current approaches to bias mitigation in AI models and calls into question the efficacy of alignment strategies employed by leading companies. As AI technologies are increasingly used in critical decision-making processes, such as sentencing in legal proceedings and evaluating asylum cases, the presence of racial biases in these models becomes a pressing concern with real-world implications. Furthermore, the authors warn against a reactive approach to bias mitigation, emphasizing the need for more comprehensive and proactive strategies to combat covert biases in AI systems.
Ultimately, the study underscores the importance of addressing racial biases in AI technologies and the need for continued research and development of more robust tools and methods to ensure fairness and equity in AI applications across various domains.
The researchers who collaborated on the study were from the Allen Institute for AI, the University of Oxford, LMU Munich, Stanford University and The University of Chicago.
read more at technologyreview.com
Leave A Comment