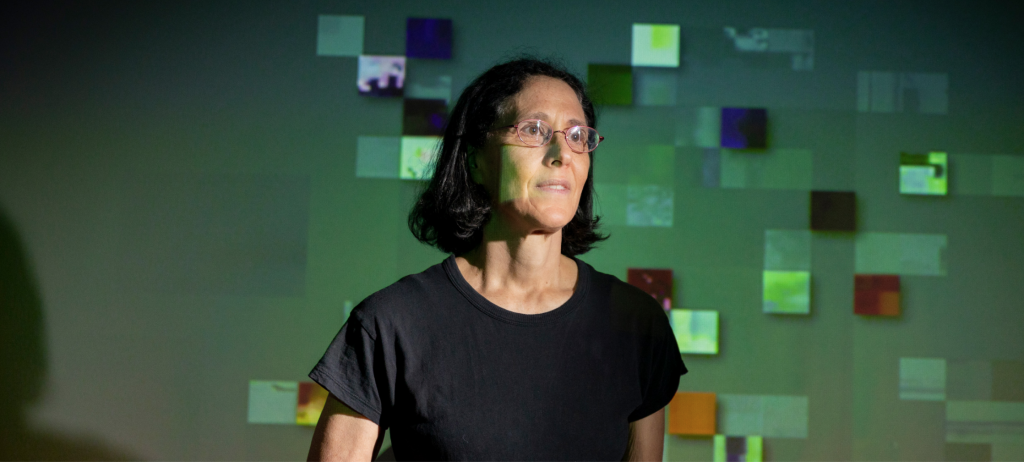
The image is of Melanie Mitchell, featured in Quanta Magazine.
Santa Fe Institute Professor Says Neural Networks Need Human Models for Thinking
A story in Quanta Magazine describes Melanie Mitchell’s thought processes on how to make AI think. The Davis professor of complexity at the Santa Fe Institute believes that algorithms need to be shaped more like the human mind if they’re ever to have real intelligence.
Analogies, which are akin to storytelling, go to the heart of human communication and thought, according to Mitchell.
“It’s understanding the essence of a situation by mapping it to another situation that is already understood,” Mitchell said. “If you tell me a story and I say, ‘Oh, the same thing happened to me,’ literally the same thing did not happen to me that happened to you, but I can make a mapping that makes it seem very analogous. It’s something that we humans do all the time without even realizing we’re doing it. We’re swimming in this sea of analogies constantly.”
The high-profile AI researcher has written two notable books. Her book Complexity: A Guided Tour (Oxford University Press) won the 2010 Phi Beta Kappa Science Book Award and was named by Amazon.com as one of the ten best science books of 2009. Her latest book is Artificial Intelligence: A Guide for Thinking Humans (Farrar, Straus, and Giroux).
Mitchell is leading SFI’s Foundations of Intelligence in Natural and Artificial Systems project, a groundbreaking collaboration involving interdisciplinary workshops over the next year examining how biological evolution, collective behavior (as in social insects such as ants), and a physical body all contribute to intelligence. When she compares human intelligence to AI, she finds AI needs more assistance to reach what are simple conclusions for humans.
“Today’s state-of-the-art neural networks are very good at certain tasks,” she said, “but they’re very bad at taking what they’ve learned in one kind of situation and transferring it to another” — the essence of analogy.
Machine learning gives algorithms hundreds or even thousands of examples, but if it doesn’t have a basis for comparison, it won’t be able to figure out complex concepts because it can’t draw from analogies, like people do, to gain from experience.
“You can show a deep neural network millions of pictures of bridges, for example, and it can probably recognize a new picture of a bridge over a river or something. But it can never abstract the notion of “bridge” to, say, our concept of bridging the gender gap. These networks, it turns out, don’t learn how to abstract. There’s something missing. And people are only sort of grappling now with that.”
The Q&A with this deep thinker is worth taking the time to absorb. According to SFI, Mitchell originated the Santa Fe Institute’s Complexity Explorer platform, which offers online courses and other educational resources related to the field of complex systems. Her online course “Introduction to Complexity” has been taken by over 25,000 students, and is one of Course Central’s “top fifty online courses of all time.”
read more at QuantaMagazine.org
Leave A Comment