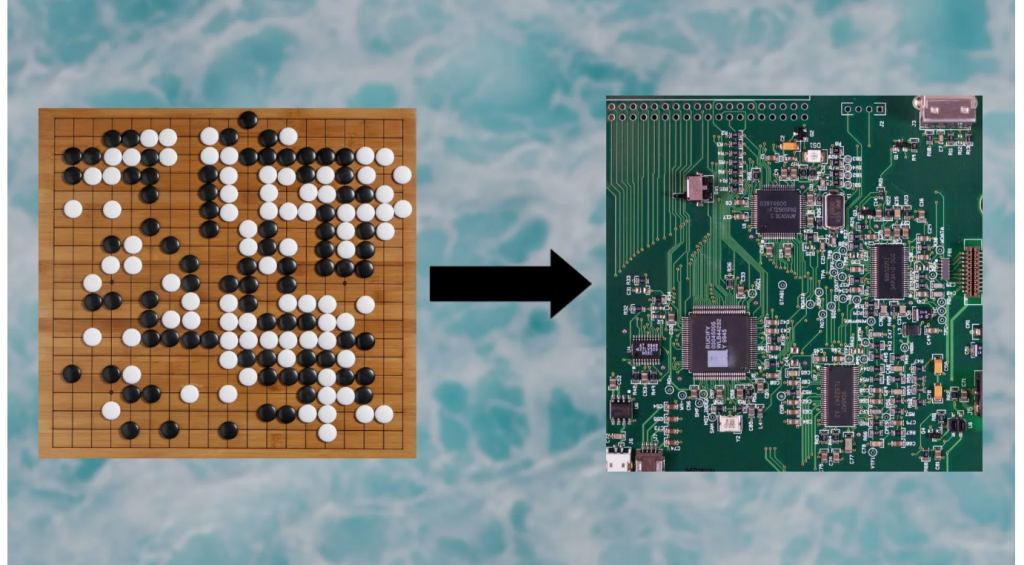
Using AI to build AI chips is far faster than using humans.
AI Computers Become Rapid Builders of AI Chips in Bid to Speed Development
Have you ever thought of your computer as a house? That is to say, have you thought about how a computer is actually built from the computer chips to the actual order in which they are placed? Chipfloor planning is the concept that an article from venturebeat.com offered this week.
“Chip floorplanning is analogous [emphasis mine] to a game with varying pieces (for example, netlist topologies, macro counts, macro sizes and aspect ratios), boards (varying canvas sizes and aspect ratios) and win conditions (relative importance of different evaluation metrics or different density and routing congestion constraints),” the researchers wrote.
The researchers mentioned are from a paper published in the peer-reviewed scientific journal Nature last week. Scientists at Google Brain introduced a deep reinforcement learning technique for floorplanning, the process of arranging the placement of different components of computer chips.
The researchers managed to use the reinforcement learning technique to design the next generation of Tensor Processing Units, Google’s specialized artificial intelligence processors.
The use of software in chip floor design is not new. But according to Google researchers, the new reinforcement learning model:
“ ‘…automatically generates chip floorplans that are superior or comparable to those produced by humans in all key metrics, including power consumption, performance and chip area. And it does it in a fraction of the time it would take a human to do so.
From six months by humans to six hours by the AI-designed chipfloor plan. Not hard to pick the right choice is it?
The Real Story Is How Intelligence Stacks Up Between AI and Humans
The interesting part of Ben Dickenson’s story is the comparison of AI to human intelligence and the order in which we both think about things.
This is the manifestation of one of the most important and complex aspects of human intelligence: analogy. We humans can draw out abstractions from a problem we solve and then apply those abstractions to a new problem. While we take these skills for granted, they’re what makes us very good at transfer learning. This is why the researchers could reframe the chip floorplanning problem as a board game and could tackle it in the same way that other scientists had solved the game of Go.
Deep reinforcement learning models can be especially good at searching very large spaces, a feat that is physically impossible with the computing power of the brain. But the scientists faced a problem that was orders of magnitude more complex than Go.
“[The] state space of placing 1,000 clusters of nodes on a grid with 1,000 cells is of the order of 1,000! (greater than 102,500), whereas Go has a state space of 10360,” the researchers wrote. The chips they wanted to design would be composed of millions of nodes.
It is a great read and filled with details that will have you scratching your own head about AI and your own path to intelligence.
read more at venturebeat.com
Leave A Comment