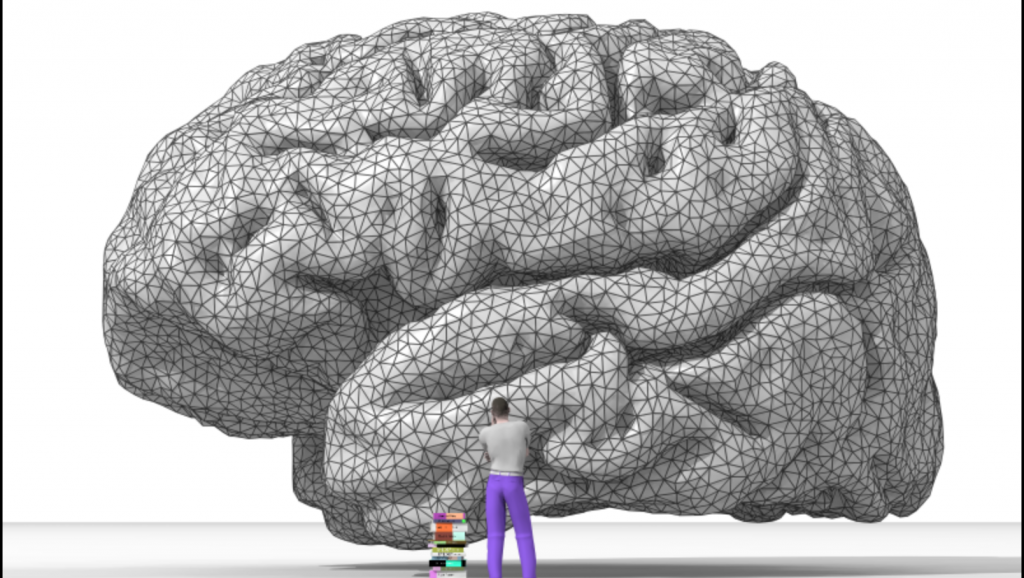
Companies can rely too much on AI for their business decisions.
Advisor Gives Executive Tips on How to Properly Use AI in Marketing, Decisions
Ahmad Alokush is the founder of technology boutique Ahmadeus, which advises C-level executives, managing directors, and fund managers on how new technology can impact their market position and overall profitability. He also has served as an expert witness in litigation concerning technology. He took time this month to write an informative and helpful piece on how companies are misusing their AI.
We found his piece at venturebeat.com and in it, Alokush writes in simple terms and rather pointed statements regarding AI and the false belief that it will solve all of our issues.
Two-thirds of CEOs surveyed last year by a major consulting firm said they will use AI even more than before for the creation of new workforce models. But are they using it to their best advantage?
Alokush writes:
As a technologist who has built platforms and worked in the major industries that employ AI often (such as FinTech and health care), I have seen first-hand what goes wrong when some of the world’s biggest companies leave their intelligence to their AI. Based on the hype around AI, it would appear that everything can be improved by sophisticated algorithms sifting through masses of data. From streamlining customer care to inventing new perfumes, and even coaching soccer teams, AI looks like an unstoppable purveyor of competitive advantage, and practically all that company executives have to do is let it loose and go have lunch (cooked by an AI Robot Chef) while they watch their company’s profits climb.
Alokush lists 4 reasons how he believes companies are making mistakes with their AI.
1. Making decisions based on the wrong data
AI is great at finding patterns in huge datasets and is efficient at predicting outcomes based on those patterns and finding uncorrelated alpha (hidden patterns in the dataset). But big problems arise when the wrong data (or outlier information) gets pulled into the dataset. In one famous example in the late 2000s, during a military coup in Thailand, the algorithm behind a major fund interpreted it as a market event, shorting a lot of Asian equities, quickly losing nine figures in dollar value. Oops.
2. Failing to train your AI properly
You can feed your AI engine all the right data and have it spit back the right answers, but until it gets tested in the wild you don’t know what it will do. Rushing to give it more responsibility than it is ready for is like sending a small child out into the real world — neither one is going to bring good results. Give your AI time to process and learn new information.
3. Ignoring the human responsibility for decisions
No matter what you program your AI to do, it will not share your human goals or bear their consequences. Thus we’ve seen early examples of AI leading early GPS users into a river, or deleting critical information to “minimize” differences in a dataset.
I’ve seen more than one startup built on the assumption that AI algorithms can learn credit approval models and replace the credit approval officer in granting/denying credit loans. However, when you are denied a credit loan, federal law requires a lender to tell you why they made that decision. Software doesn’t really make decisions (it just identifies patterns) and isn’t responsible for decisions; humans are. Since federal law agrees that humans are responsible for credit decisions, many of these startups burned through venture capital funds and then could not legally launch to customers because the AI they developed was inherently biased. When it denied loans, no human could adequately explain why the denial occurred.
4. Overvaluing data
Some data simply can’t be used to build anything useful. One of our clients that failed at using AI was a popular medical diagnosis platform with its own data lake and a broad array of datasets. The company that owned it had acquired another platform with its own array of siloed datasets. The executives wanted to glean some insight into the jumble of disconnected datasets and needed help with onboarding potential customers. The problem was that these datasets were describing different medical issues/profiles, and trying to find common denominators of any real value was not possible. Despite all the compiled information, working with this client’s data was like having lego pieces that didn’t actually connect. Just because they are alike in many respects does not mean that you can build a castle out of them. After consulting with the client, we recommended they not do the project.
So, here at Seeflection.com, we are solidly behind AI and the amazing things it is making possible in our modern world. However, there is still much to learn and much to apply to the use of AI and Machine Learning.
read more at venturebeat.com
Leave A Comment