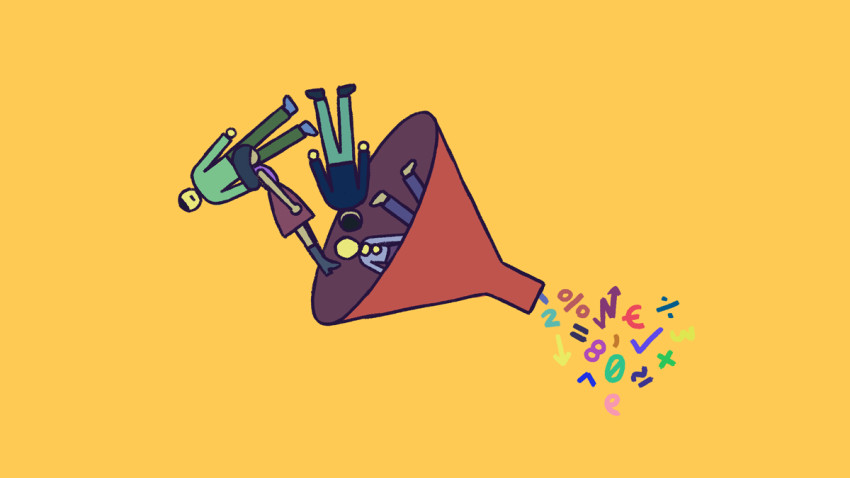
Art created for Harvard Business Review by Vincent Tsui
Machine Learning Brings Data-Driven Approach for B2B
AI-based B2B⎯Business to Business marketing via data mining⎯lags far behind the Business to Consumer model, but that may soon change thanks to new AI-based algorithms from Google and an analytic approach from Everstring Technology, according to an article in the Harvard Business Review.
“Neural networks and “deep learning” algorithms along with other machine learning methods enable data scientists to mine the gold in digital formats. These AI-based methods involve advanced search techniques that identify, categorize, and gather user-defined data elements corresponding to search criteria. For example, considerable business description information exists on LinkedIn. But how can organizations analyze each profile on the business network? Well-designed AI-based algorithms are the key to extracting key information from LinkedIn. These more structured data resources then provide the platform for yet another application of AI-based algorithms, where the focus is on identifying patterns in data that ultimately provide the basis for predictive sales and marketing models.”
Everstring Technology is deploying machine learning to create a micro-categorization scheme that not only draws from descriptive sources such as business websites, employee digital footprints and expert sources in the B2B space, but also uses models derived from a company’s internal information.
For instance, Autodesk uses an “Enterprise Business Agreement Model” developed through Everstring’s predictive analytics to identify which executives within a large customer organization are most likely to seek an enterprise-level agreement with the company. Autodesk then develops an overall account potential model. This allows the sales force to work from ranked recommendations and “raw scores” derived from the models. The sales team has found the analyses to be valuable, according to Matthew Stevens, Autodesk’s Sales Insights Manager.
“It’s early to judge the exact payoff, but it’s difficult to imagine making a recommendation without these insights,” Stevens said. “We are challenged to respond to all the questions about accounts and scores, but at least we have data to support our recommendations now.”
Additionally, Stevens expects the model to have a global impact because of the difficulty in finding data on European and Asian companies, which have more restrictive privacy regulations and language difference. The authors of the article, Stephan Kudyba and Thomas H. Davenport, say it’s just the start, but machine learning may make it possible for B2B to catch up to B2C marketing quickly.
“The amount and quality of data on businesses may not yet approach that for consumers, but there is considerable progress being made in achieving parity.”
Read more at Harvard Business Review
Leave A Comment