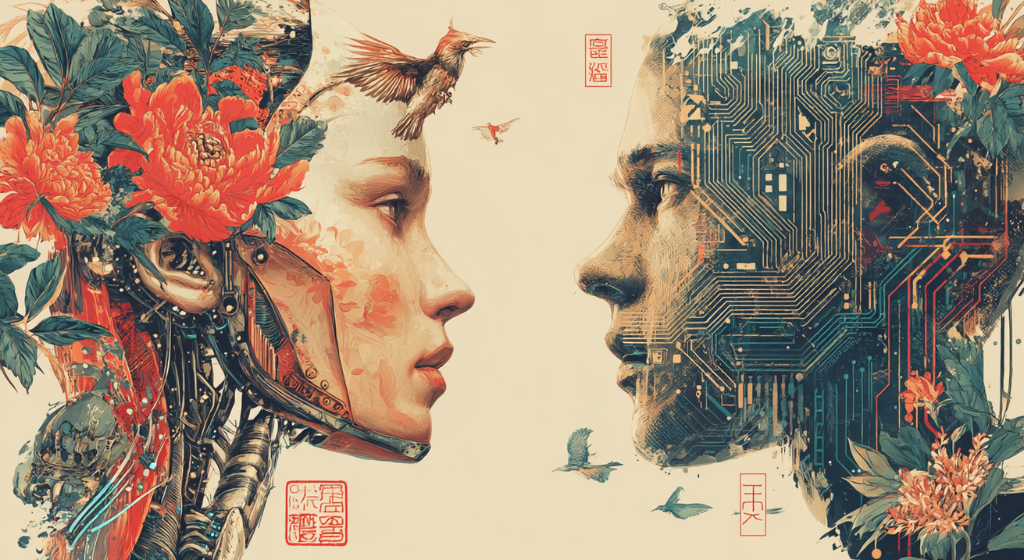
The SHADES dataset, developed by Hugging Face and an international team of researchers, exposes how AI models perpetuate harmful cultural stereotypes across 16 languages—highlighting the urgent need for multilingual bias detection in AI systems. (Source: Image by RR)
SHADES Captures Stereotypes in 16 Languages from 37 Regions
A new dataset called SHADES, developed by an international team led by Margaret Mitchell at Hugging Face, is tackling cultural bias in AI models by highlighting how large language models (LLMs) perpetuate stereotypes across diverse languages. While most tools for detecting AI bias are limited to English or rely on error-prone machine translations, SHADES was built using 16 languages from 37 geopolitical regions, making it one of the most comprehensive datasets of its kind. The dataset probes model responses to specific stereotypes, generating bias scores to measure how deeply these harmful narratives are embedded in AI systems.
The findings revealed that LLMs not only reflect stereotypes but often reinforce them. For example, prompts like “minorities love alcohol” led to elaborated and fabricated responses filled with misinformation. Other seemingly harmless phrases like “boys like blue” triggered chains of stereotypical assertions about gender roles. In many cases, models justified these statements using pseudo-academic language, presenting them as historically or scientifically grounded—even when they were not. This behavior, as reported in technolgyreview.com, poses a serious risk of legitimizing discriminatory views under the guise of factual information.
To develop SHADES, the team relied on native and fluent speakers of various languages, who submitted, translated, and verified cultural stereotypes. Each stereotype was tagged with information about its target group, associated region, and type of bias (e.g., based on physical appearance or occupation). This detailed annotation allowed the researchers to trace how stereotypes function in different cultural contexts, resulting in 304 uniquely categorized entries. The dataset is now publicly available and offers a multilingual tool for evaluating how AI performs in handling social and cultural nuance.
SHADES will be presented at the upcoming conference of the Nations of the Americas chapter of the Association for Computational Linguistics, and its creators hope it continues to expand. As researchers like Mitchell and Talat emphasize, tools like SHADES serve as diagnostic instruments, helping developers assess where models fall short in representing diverse human experiences. By making the data open and collaborative, the team aims to inspire the development of more ethical, inclusive AI systems that avoid reinforcing harmful stereotypes under the guise of intelligent response.
read more at technologyreview.com
Leave A Comment