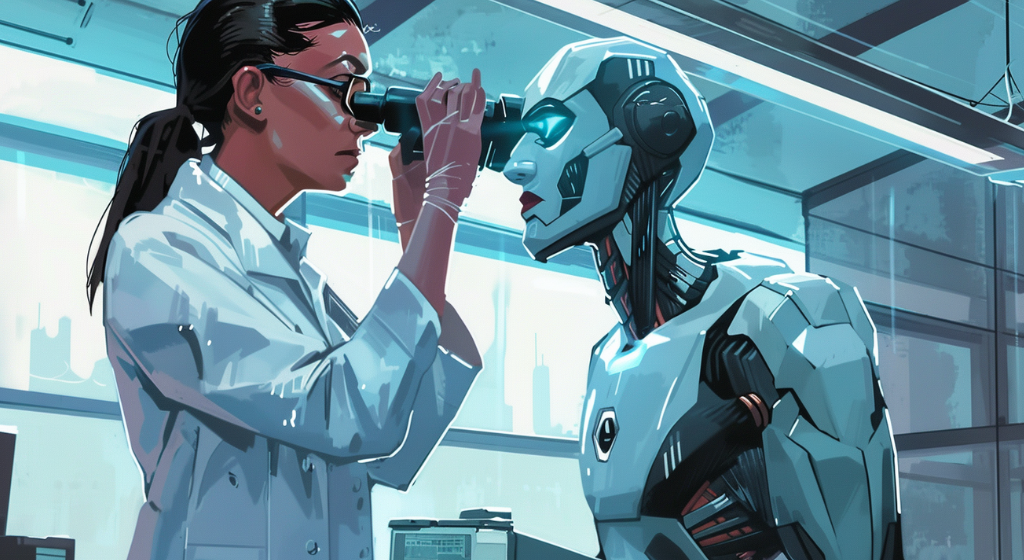
AI does not have peripheral vision, unlike humans, but equipping computer vision models with this ability could help them detect approaching hazards more effectively or predict whether a human driver would notice an oncoming object. (Source: Image by RR)
AI Still Falls Short of Human-Level Performance in Peripheral Object Detection
Researchers at MIT have made significant strides in enhancing the peripheral vision capabilities of AI models, bringing them closer to human-like vision and potentially improving driver safety and our understanding of human behavior. Unlike humans, AI lacks peripheral vision, which limits its ability to detect objects outside the central field of view. To address this gap, MIT researchers developed a novel image dataset that simulates peripheral vision in machine learning models. This dataset allows models to better detect and recognize objects located off to the side or in the corners of a scene.
The researchers utilized a modified technique called the texture tiling model to transform images, mimicking the information loss that occurs in human peripheral vision. According to a report in news.mit.edu, this approach generated a vast dataset of transformed images, representing the loss of detail in the periphery. Training computer vision models with this dataset resulted in improved performance in detecting objects, although the models still fell short of human-level performance. Interestingly, the size of objects and visual clutter in a scene did not significantly impact the AI’s performance, highlighting differences in how humans and models perceive and process visual information.
Despite the progress made, challenges remain in bridging the gap between human and machine perception in the periphery. The researchers aim to further investigate these differences to develop AI models that can better predict human performance in peripheral vision tasks. Such models could have applications in driver assistance systems and other real-world scenarios where detecting peripheral objects is crucial for safety.
Additionally, the researchers hope to encourage further computer vision research using their dataset, contributing to advancements in AI perception and understanding of human vision. This work not only sheds light on the complexities of peripheral vision but also underscores the importance of interdisciplinary collaboration between neuroscience, computer science, and engineering in advancing AI technologies.
read more at news.mit.edu
Leave A Comment