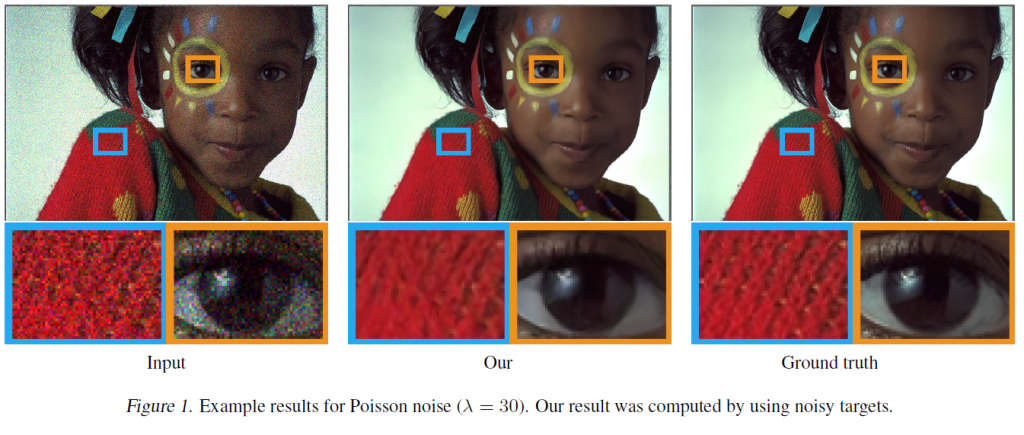
AI Cleans Up Noisy Images Sans Training Data
NVIDIA announced this month that the company’s researchers have achieved impressive results in a joint study with two universities using deep learning to de-noise grainy images with a stunning degree of clarity—using only noisy or corrupted input image data. Conducted alongside researchers from Aalto University and MIT, NVIDIA published Noise2Noise: Learning Image Restoration without Clean Data (pdf) and released a news post about the results of the study on the company’s blog.
The bane of digital photography and videography and a concern in similar fields such as radiology, anyone who’s tried to record a candlelit birthday, concert video, or photo of the Milky Way has undoubtedly faced dismay at the inevitably grainy, muddled, noise-ridden results. A by-product of increased light sensitivity, image noise is most prevalent in limited light exposure conditions, such as in high-speed or low-light photography necessitating increased sensor ISO, or sensitivity.
While larger and more advanced cameras sensors have improved markedly with the latest generations of sensor technology, noise reduction is still a time-consuming and costly process for image and video post-production. While automated image enhancement and automated noise reduction is no new trick, NVIDIA’s AI approach is unique not only in the quality of its results, but also in the research team’s breakthrough approach.
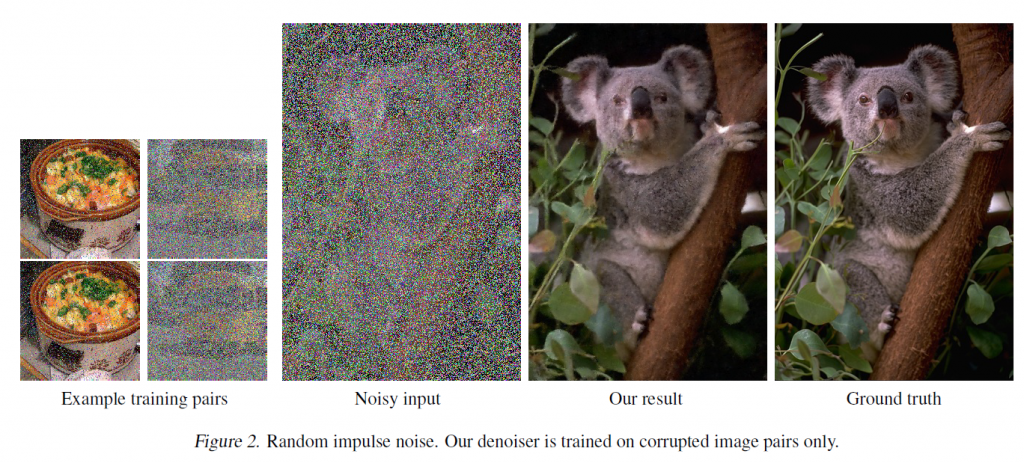
From a sample of noisy images (far left), the AI can de-noise even extremely corrupted images (left) into results that are remarkably accurate when compared to unaltered “ground truth” images. Via NVIDIA.
Noting that their AI is “on par with state-of-the-art methods that make use of clean examples — using the same training methodology, and often without appreciable drawbacks in training time or performance,” the team observed that “under certain common circumstances, it is possible to learn to restore signals without ever observing clean ones, at performance close or equal to training using clean exemplars.” In other words, the team used similar methods to previous attempts to de-noise images using neural networks, but without training their network on noise-free “clean” images for the AI to compare the noisy photos to, obviating the need for “potentially strenuous collection of clean data”.
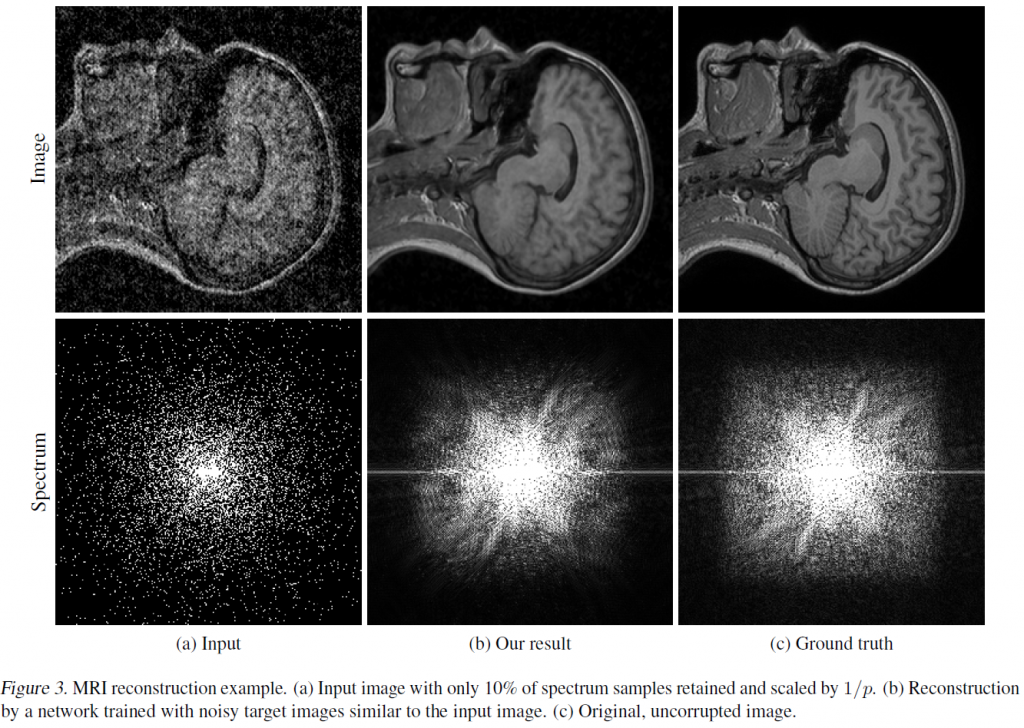
The same technology being tested to clean up corrupted MRI images.
According to the study, the team’s trained AI only requires the sampling of two noisy images to de-noise a target image, with the resultant image stunningly clear and almost as clear as the unadulterated “ground truth” clean images.
The Santa Clara, CA-based GPU giant and rising AI star NVIDIA has shifted from being an eminent producer of GPUs for gamers, media professionals, and others requiring top-notch graphics cards towards a more AI-centric business model with the advent of GPU-powered AI networks. Employing precisely this technology—NVIDIA’s Tesla P100 GPUs, namely—with a cuDNN-enhanced TensorFlow framework, the team trained their system on 50,000 images in the ImageNet validation set, using only noisy or otherwise corrupted images.
In addition to cleaning up video or photo noise in post-processing, the technology can also be used for other purposes. The team suggests that the technology could prove a boon to radiology and other medical applications where such neural networks could clean and enhance images of potentially life-saving importance.
In another potential application the paper hints at is watermark removal. The AI is so adept at removing noise that it even aces situations where the “noise” in question is strings of text overlaid on images, meaning that even advanced, intricate watermarks on copyrighted photos or video might soon be no match for content thieves taking similar machine learning approaches.
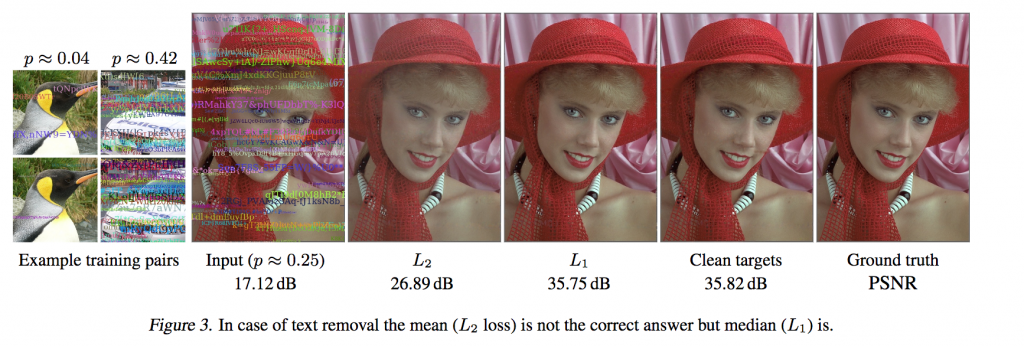
The AI can remove the text (images at left) with remarkable acuity compared to the unaltered image (far right)—all accomplished only with the corrupted image as input.
While the results of the researchers’ AI reach impressive levels of accuracy and clarity—especially given the lack of clean input data—the AI is still an imperfect solution compared to techniques to avoid noise in image acquisition, as data is inevitably lost in any attempt to enhance a noisy, blurry, or otherwise less-than ideal image. “There is no free lunch,” say the authors: “we cannot learn to pick up features that are not there in the input data – but this applies equally to training with clean targets.”
The researchers will join other researchers from academia and industry to present their findings this month at the 35th International Conference on Machine Learning (ICML) in Stockholm, Sweden.
Leave A Comment