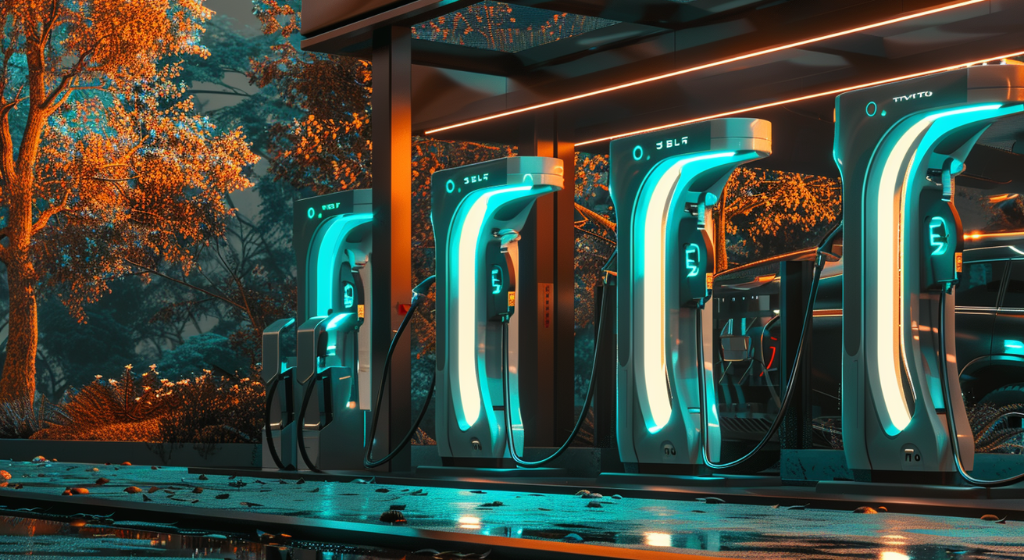
The shift to electric vehicles (EVs) signifies a pivotal industry transformation towards zero emissions and tackles environmental and health issues from traditional vehicles, increasing the demand for adept charging station management and necessitating accurate power consumption forecasts. (Source: Image by RR)
Machine Learning Powers the Future of Electric Vehicle Charging Stations
A recent study published in Scientific Reports has shed light on the benefits of utilizing machine learning to forecast power consumption and potential income at electric vehicle charging stations (EVCS). Researchers have developed a time series model to accurately predict the power usage and revenue generation of EVCS, employing statistical techniques to analyze historical data. As reported in azoai.com, this approach aims to optimize resource management and operations by identifying revenue trends and managing the power supply efficiently.
The shift toward electric vehicles (EVs) necessitates advanced management of EV charging stations to meet growing demand while avoiding grid overload. Accurate forecasting of power consumption is essential for planning the required number of charging stations and ensuring their efficient operation. Past attempts to forecast this demand through various methods, including simple statistical models and machine learning, have fallen short, highlighting the need for more sophisticated forecasting techniques.
The researchers examined power consumption at charging stations using three different time series models: auto-regressive moving average (ARMA), auto-regressive integrated moving average (ARIMA), and seasonal auto-regressive integrated moving average (SARIMA), with the latter proving most effective in predicting power demand and income generation. The study analyzed data from public charging stations in Colorado and a station in Kerala, India, to understand power usage patterns and inform resource allocation based on different vehicle types. This analysis is crucial for developing pricing strategies that maximize profits while staying competitive.
The findings indicated that SARIMA models were superior in forecasting power demand, with DC charging stations showing higher electricity usage than AC stations, attributed to their faster charging capabilities. This research highlights the importance of precise electricity demand prediction for EVCS, aiding utility companies and charging network operators in optimizing operations and making informed decisions regarding infrastructure planning and pricing strategies. The study acknowledges limitations such as data collection constraints and suggests future research directions, including integrating time series models with machine learning techniques and exploring dynamic pricing strategies.
read more at azoai.com
Leave A Comment