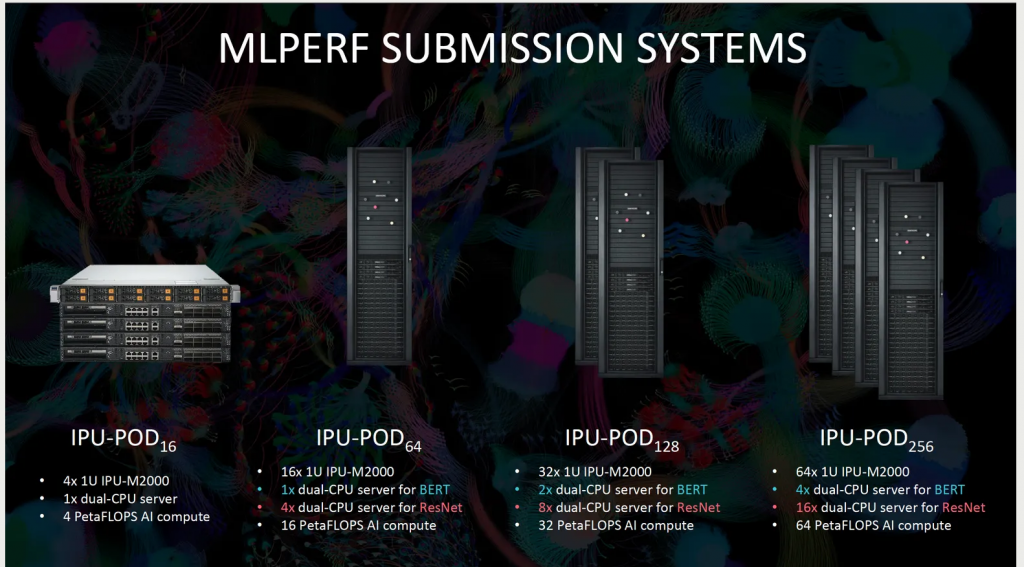
Graphcore brought its bigger systems out to play and was able to boost GPUs. (Source: Graphcore)
Systems Using A100 GPUs Have Increased More Than 5-fold In The Last 18 Months-Nvidia
Remember when computers were young? Remember how many of the things we take for granted today on our tablets and with our smartphones, were still clunky and algorithms were learning how to learn? Machine learning would take forever. Well back around 1965 a new term came into being and it involved transistors. It was called Moore’s Law.
Moore’s law is a term used to refer to the observation made by Gordon Moore in 1965 that the number of transistors in a dense integrated circuit (IC) doubles about every two years. Moore’s law isn’t really a law in the legal sense or even a proven theory in the scientific sense (such as E = mc2).
For several years computers followed Moore’s Law pretty closely. Their CPUs and the software of the time would essentially double every two years.
Then came AI into the equation and the numbers show that it’s blown Moore’s Law out of the water.
The gains to AI training performance since MLPerf benchmarks began “managed to dramatically outstrip Moore’s Law,” says David Kanter, executive director of the MLPerf parent organization MLCommons
The days and sometimes weeks it took to train AIs only a few years ago was a big reason behind the launch of billions of dollars worth of new computing startups over the last few years—including Cerebras Systems, Graphcore, Habana Labs, and SambaNova Systems. In addition, Google, Intel, Nvidia, and other established companies made their own similar amounts of internal investment (and sometimes acquisition).
With the newest edition of the MLPerf training benchmark results, there’s clear evidence that the money was well spent.
How Much Faster Can It Be?
But after decades of intense effort and hundreds of billions of dollars in investment, look how far we’ve come! According to Research Gate, if you’re fortunate enough to be reading this article on a high-end smartphone, the processor inside it was made using technology at what’s called the 7-nanometer node. That means that there are about 100 million transistors within a square millimeter of silicon. Processors fabricated at the 5-nm node are in production now, and industry leaders expect to be working on what might be called the 1-nm node inside of a decade.
Yes, things have gotten much quicker. AI is making training algorithms so much faster, it is hard to put into layman’s terms. It is the difference between a propeller plane and a modern jet fighter. The jump is that dramatic.
An article in spectrum.ieee.org was written by Samuel k Moore and this Mr. Moore explains what several of the companies listed above found out when they tested some of their latest algorithms with a company called ML Commons. The testing part was run through the benchmarks of MLPerf which is part of ML Commons.
Overall improvements to the software as well as processor and computer architecture produced a 6.8-11-fold speedup for the best benchmark results. In the newest tests, called version 1.1, the best results improved by up to 2.3 times over those from June.
For the first time, Microsoft entered its Azure cloud AI offerings into MLPerf, muscling through all eight of the test networks using a variety of resources. They ranged in scale from 2 AMD Epyc CPUs and 8 Nvidia A100 GPUs to 512 CPUs and 2048 GPUs. Scale clearly mattered. The top range trained AIs in less than a minute while the two-and-eight combination often needed 20 minutes or more.
If you are not well versed in these numbers and they make no sense to you, the article explains in detail what the results for each tech company mean and just what algorithms were tested.
But the bottom line is this, AI Is making advances so much faster than even the best minds anticipated. And believe it or not, that is a good thing.
read more at spectrum.ieee.org
.
Leave A Comment