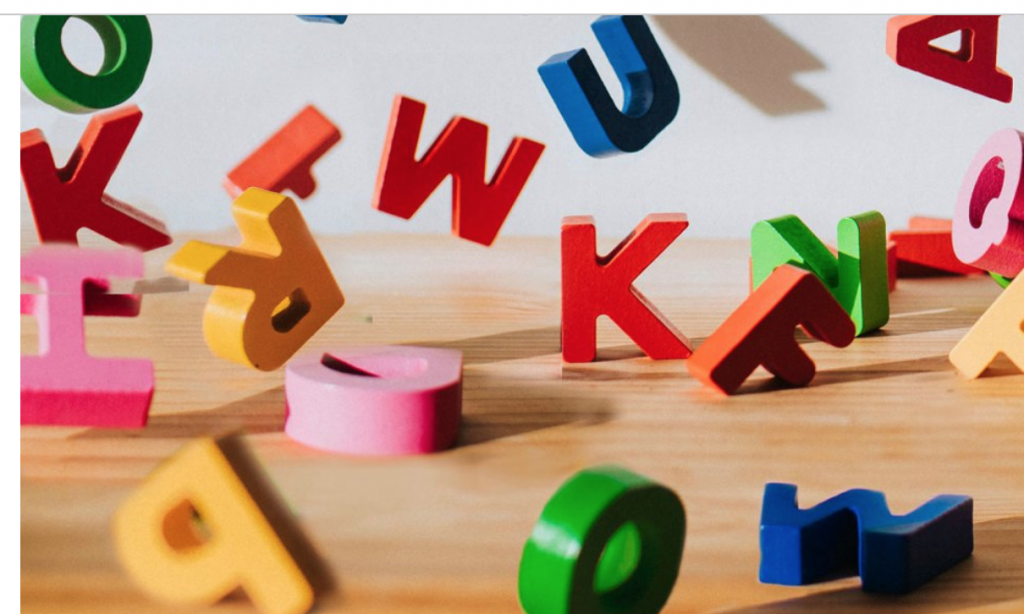
Summarizing AI papers just got a big assist from Semantic Scholar.
Semantic Scholar Summarizes Scientific Papers to Help Researchers Find Data
The letters TL:DR stand for “too long didn’t read.” And in the case of researching millions of pages of AI data, that symbol can be becoming up far too often. Say hello to Semantic Scholar.
A new AI model for summarizing scientific literature can now assist researchers in wading through and identifying the latest cutting-edge papers they want to read. On November 16, the Allen Institute for Artificial Intelligence (AI2) rolled out the model onto its flagship product, Semantic Scholar, an AI-powered scientific paper search engine. It provides a one-sentence tl;dr (too long; didn’t read) summary under every computer science paper (for now) when users use the search function or go to an author’s page. The work was also accepted to the Empirical Methods for Natural Language Processing conference this week.
Karen Hao has an article in technologyreview.com this week that introduces this time-saving AI to the AI community at large.
How They Did It
Using AI to summarize text has been a popular natural-language processing (NLP) problem using two general approaches . One is “extractive,” which seeks to find a sentence or set of sentences from the text verbatim that captures its essence. The other is called “abstractive,” which involves generating new sentences. While extractive techniques were often used due to the limitations of NLP systems, advances iin recent years have made the abstractive one far better.
“AI2’s abstractive model uses what’s known as a transformer—a type of neural network architecture first invented in 2017 that has since powered all of the major leaps in NLP, including OpenAI’s GPT-3,” the story explains. “The researchers first trained the transformer on generic bodies of text to establish its baseline familiarity with the English language. This process is known as “pre-training” and is part of what makes transformers so powerful. They then fine-tuned the model—in other words, trained it further—on the specific task of summarization.”
Also, the AI tool can achieve an extreme level of compression. Scientific papers used average 5,000 words; the tool averages 21 words in summaries or 238 times the paper’s size. Reviewers also judged the model’s summaries to be more informative and accurate than previous methods.
Next Steps
AI2 designers are already working to improve the model, says Daniel Weld, a professor at the University of Washington and manager of the Semantic Scholar research group. For instance, they plan to train the model to handle more than just computer science papers. Due to the training process, they’ve also found that the tl;dr summaries sometimes overlap too much with the paper title. They plan to update the model’s training process to penalize overlaps so it learns to avoid repetition.
In the long-term, the team will also work summarizing multiple documents at a time, which could be useful for researchers entering a new field or perhaps even for policymakers wanting to get quickly up to speed.
“What we’re really excited to do is create personalized research briefings,” Weld says, “where we can summarize not just one paper, but a set of six recent advances in a particular sub-area.”
read more at technologyreview.com
Leave A Comment