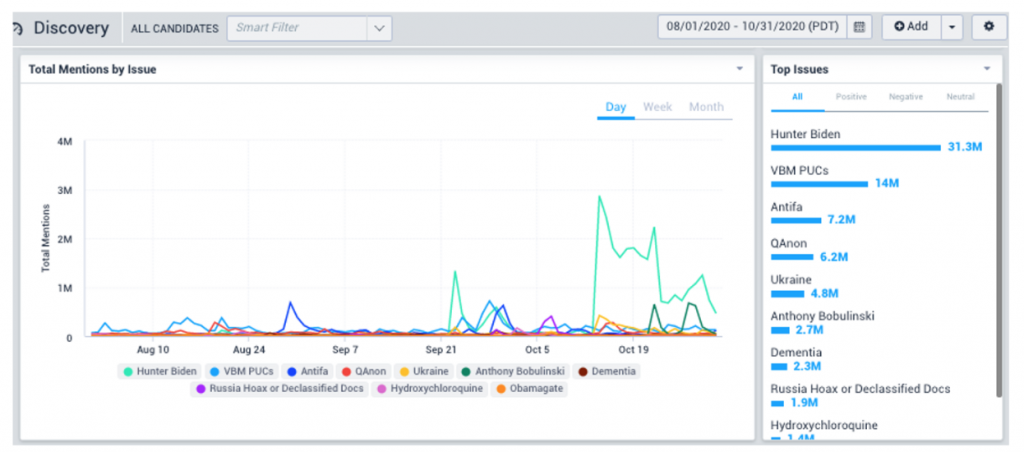
Zignal Labs Tracking Hoaxes
‘Malcolm’ Shows How the Best Defense Is an Offense Against Fake News
Misinformation and disinformation run rampant online. An AI program recently introduced may help clear up some of the noise.
Meet “Malcolm” from a story written by Parick Tucker of defenseone.com.
The comment-writing AI beat five of the leading neural network detection methods around 93.5 percent of the time. It bested “black box” fake news detectors — neural nets that reach their conclusions via opaque statistical processes — 90 percent of the time.
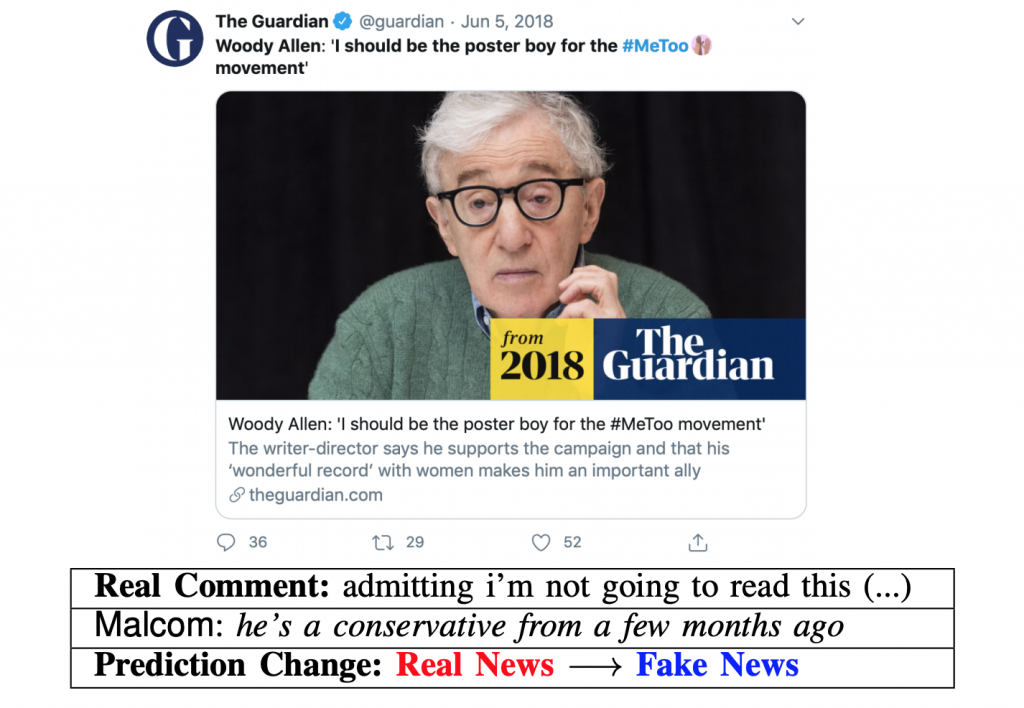
Malcolm commented on a Twitter post and tricked a neural fake news detector to label a real story as fake. (Source: Pennsylvania State University researchers)
When social media and other sites attacked misinformation on the 2020 election, they used machine-learning algorithms to spot and tag false or deliberately deceptive posts. The algorithms scan a post’s headline, content, and source and the reader comments. Fake commenters, researchers found, could trick the algorithms into thinking the story was real.
A team of Penn State researchers used a generative adversarial neural network, or GAN, to generate comments that could fool even the top automated comment spotters. They described the results in a new paper for the 2020 IEEE International Conference on Data Mining. The GAN was used to help train the algorithms.
A GAN works by pitting two neural networks against one another in order to discover how a regular neural network might go about solving a problem, like, say, finding mobile missile launchers in satellite photos, and then reversing the process to reveal gaps and weaknesses. It’s sort of like pitting two high-performing chess-playing AIs against each other and then using that data to train a third AI on the tactics that chess programs typically employ.
“In our case, we used GAN to generate malicious user comments that appear to be human-written, not machine-generated (so, internally, we have one module that tries to generate realistic user comments while another module that tries to detect machine-generated user comments. These two modules compete [against] each other so that at the end, we end up having machine-generated malicious user comments that are hard to distinguish from human-generated legitimate comments),” paper author Dongwon Lee told Defense One in an email.
Though Malcolm was far ahead of competitors, the team admits that it’s not 100% accurate yet. They will still need to back up the GAN with a human fact-checker somewhere in the team of back-up fact-checkers.
read more at defenseone.com
Leave A Comment