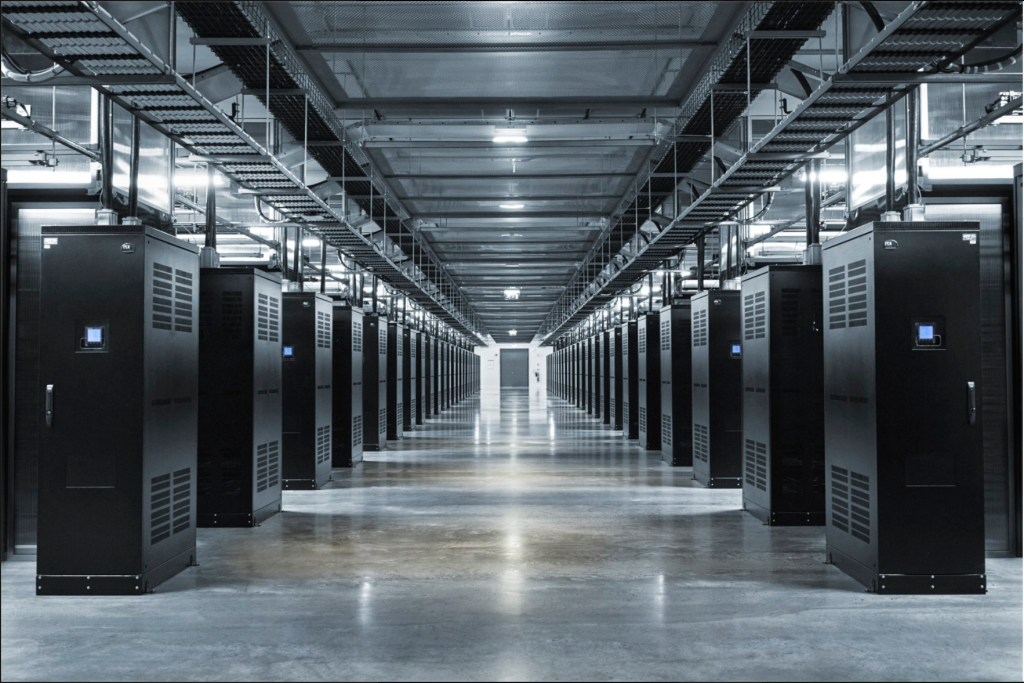
A Facebook server farm operates in thousands of square feet in a cold climate in Sweden 70 miles south of the Arctic circle. It uses 40% less power than a traditional server farm. AI developers face similar issues. (Source: Mark Zuckerberg on Facebook)
GPT-3 Represents Latest in Machine Learning, Energy Consumption
“Human brains can do amazing things with little power consumption,” as AI researcher Siva Reddy put it. “The question is how can we build such machines.”
The stunning conclusion of an article on forbes.com is that certain AI models leave a massive carbon footprint. Written by Rob Toews, a venture capitalist at Highland Capital Partners, the story brings into focus a growing problem in AI development.
For instance, earlier this month OpenAI announced it had built the biggest AI model in history. This eye-opening large model, known as GPT-3, is an impressive technical achievement. Yet it also is a monster of consumption.
Modern AI models consume a tremendous amount of energy, and these energy requirements are growing at a breakneck rate. In the deep learning era, the computational resources needed to produce the next great AI model has on average doubled every 3.4 months. That comprises a 300,000x increase between 2012 and 2018. GPT-3 is just the latest example of this skyrocketing growth.
“The bottom line: AI has a meaningful carbon footprint today, and if industry trends continue it will soon become much worse,” Toews writes. “Unless we are willing to reassess and reform today’s AI research agenda, the field of artificial intelligence could become an antagonist in the fight against climate change in the years ahead.
Toews explains that GPT-3 is comprised of 175 billion parameters, which can only be comprehended by compare it to its predecessor model, GPT-2, with only 1.5 billion parameters. Resultingly, Toews writes, “While last year’s GPT-2 took a few dozen petaflop-days to train—already a massive amount of computational input—GPT-3 required several thousand.”
The problem with relying on ever-larger models to drive progress in AI is that building and deploying these models entails a tremendous amount of energy expenditure and thus carbon emissions. As Toews writes:
“The ‘bigger is better’ ethos that currently dominates the AI research agenda threatens to inflict major environmental damage in the years ahead. Thoughtful, bold change is needed to set the field of artificial intelligence on a more sustainable and productive trajectory.”
Toews points out there was little improvement on the data found on several AI models, in spite of the amount of energy required to run them.
In a widely discussed 2019 study, a group of researchers led by Emma Strubell estimated that training a single deep learning model can generate up to 626,155 pounds of CO2 emissions—roughly equal to the total lifetime carbon footprint of five cars. As a point of comparison, the average American generates 36,156 pounds of CO2 emissions in a year.
Other simple solutions like reducing wasted resources and using a carbon neutral cloud provider can help reduce AI’s carbon footprint in the near term, Toews writes. Other means are more efficient hyperparameter search methods, reducing the number of unnecessary experiments during training and employing more energy-efficient hardware.
But AI developers ultimately need to find a “fundamental long-term shift” to reduce their carbon footprint.
It is a fascinating article that zeroes in on what progress is actually costing our planet.
read more at forbes.com
Leave A Comment