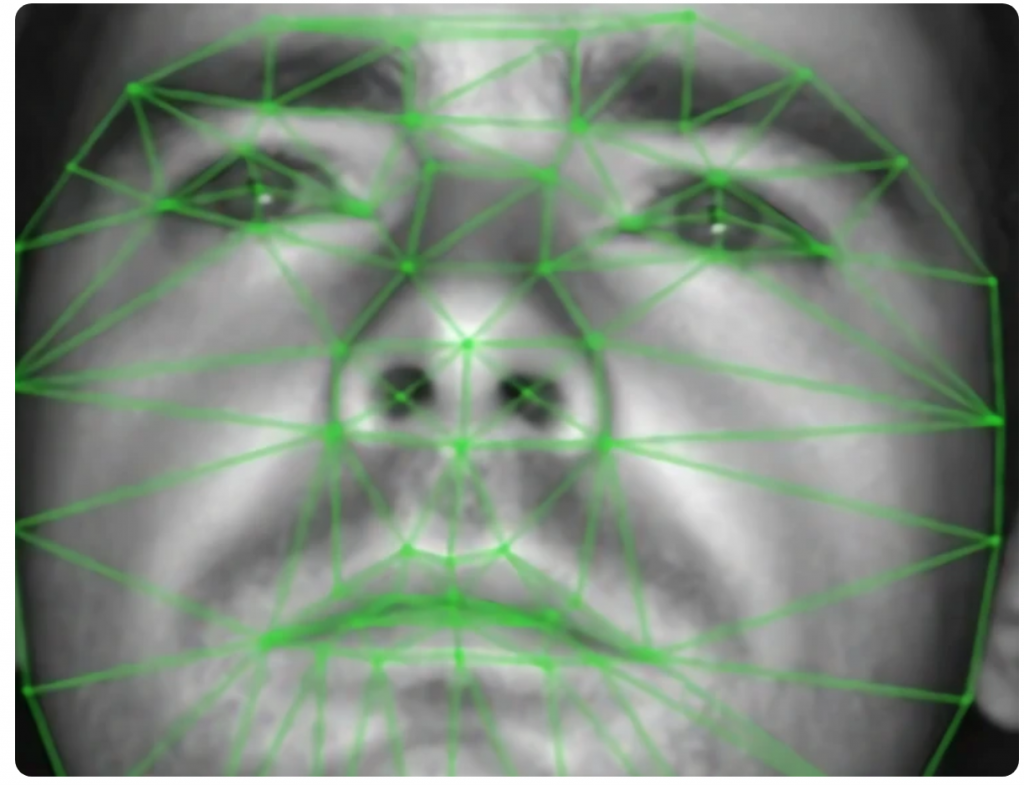
LIBS makes lip reading more accurate.
Some Pose Questions about Ethics, Profitability of Lip Reading
Major League Baseball and the Houston Astros are investigating new allegations that the Texas team illegally used electronic equipment to steal opponents’ pitching signs during their championship season two years ago. This problem began as soon as baseball started using signals to tell pitchers whether to throw a fastball or a curveball. Now it may become even easier to see what the manager or line coach is saying.
Kyle Wiggers of venturebeat.com wrote about AI’s ability to read lips from videos, which he explains is not a brand new idea:
“AI and machine-learning algorithms capable of reading lips from videos aren’t anything out of the ordinary, in truth. Back in 2016, researchers from Google and the University of Oxford detailed a system that could annotate video footage with 46.8% accuracy, outperforming a professional human lip-reader’s 12.4% accuracy.”
In pursuit of a higher performing system, researchers at Alibaba, Zhejiang University, and the Stevens Institute of Technology devised a method dubbed Lip by Speech (LIBS), which uses features extracted from speech recognizers to serve as complementary clues. They say it manages industry-leading accuracy on two benchmarks, besting the baseline by a margin of 7.66% and 2.75% in character error rate.
Wiggers describes how this could help a wide range of people. LIBS and other solutions like it could help those hard of hearing to follow videos that lack subtitles. An estimated 466 million people globally suffer from disabling hearing loss, about 5% of the world’s population. By 2050, the number could rise to over 900 million, according to the World Health Organization. LIBS distills useful audio information from videos of human speakers at multiple scales, including at the sequence level, context level, and frame level. It then aligns this data with video data by identifying the correspondence between them (due to different sampling rates and blanks that sometimes appear at the beginning or end, the video and audio sequences have inconsistent lengths), and it leverages a filtering technique to refine the distilled features.
Both the speech recognizer and lip reader components of LIBS are based on an attention-based sequence-to-sequence architecture, a method of machine translation that maps an input of a sequence (i.e., audio or video) to an output with a tag and attention value.
It’s a technical article containing complex information, but could be of use⏤especially for scouts seeking the Washington Nationals pitch signals from this year’s World Series.
read more at venturebeat.com
Leave A Comment