Researcher Explains Spooky Costumes as Showing AI Flaws
Halloween costumes can be incredibly predictable some years: the latest super hero or politician. But that’s not the case where AI is concerned. An algorithm recently concocted a strange brew of costume ideas based on input, data and using machine learning.
According to an opinion piece in The New York Times written by AI researcher Janelle Shane, who writes the blog AIweirdness.com, training an algorithm called “textgenrnn” led to some freaky results that most people wouldn’t consider as options for a costume. The exercise is emblematic of how AI comes to strange conclusions based on data. She asserts that we shouldn’t trust AI to make important decisions for us without oversight.
The dataset the AI used included 7,182 costumes that already exist. Its suggestions for new costumes or predicting what costumes are to come were, well, weird.
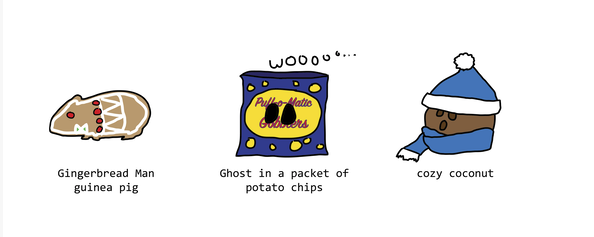
Examples of AI costume designs from AIweirdness.com.
Some of AI’s suggestions included “Ruth Bader Hat Guy,” “Alien Chestman,” “The Crayon,” and “Pirate The Wild Thor.” As it trained, it developed slightly more usable concepts, such as “Deatheater,” “Dragon Ninja,” and “Cat Witch.”
Shane said the “spooky” nature of AI is that it isn’t possible to know exactly how it determines that an idea is viable. One word it decided to use in multiple iterations was “Sexy.” That would seem a strange decision for an AI, except that so many costumes for women have that title on them, including “Sexy Nurse,” “Sexy Pirate,” “Sexy Schoolgirl” and “Sexy Supergirl.
A story in fastcompany.com examined the exercise, and commented:
“Want to see all the costumes the AI has on offer? The New York Times article includes an interactive where you can click through to see a ton of different ideas–many of which are pretty terrible. My first few clicks yielded “sexy cthulhu,” “princesseon,” and “the rcdonagall.” One of my favorites though? “Space lord.” All I’d need is a glittery jumpsuit, a crown, maybe throw in a lightsaber, and I’m done.”
Shane concludes in her article that AI is not ready for prime time in a lot of areas, including landing an airplane, making parole decisions and job hiring. She offered examples of how this has and can go wrong.
“For example, an algorithm that sees hiring decisions biased by race or gender will predict biased hiring decisions, and an algorithm that sees racial bias in parole decisions will learn to imitate this bias when making its own parole decisions. After all, we didn’t ask those algorithms what the best decision would have been. We only asked them to predict which decisions the humans in its training data would have made,” Shane wrote.
She’s done a TED Talk podcast on AI. Also, she is promoting her book “You Look Like A Thing and I Love You!” about AI weirdness, a title that AI generated, of course.
Leave A Comment