Dual Neural Network Shows 70% IDing Accuracy in Series of Tests
Researchers have proven that consumers are heavily influenced by online reviews⏤so it’s vital to find the positive reviews generated by computers or by people who fake them. Scientists have devised a two-part neural network that may solve the problem.
Researchers at the Hartman Group and the University of Washington developed a “spamGAN” or Generative Adversarial Network that can tell the difference between computer-generated and user fakes or “unrealistic” reviews and real reviews. The network has generators that produce samples and “discriminators” that use a body of annotated and unannotated data to determine which are generated and which are real, according to a story in Venturebeat.com. The research was published on Arvix.com, prior to print publication.
“Opinion spam is a widespread problem in ecommerce, social media, travel sites, [and] movie review sites,” the paper’s authors write. “Opinion spammers try to exploit such financial gains by providing spam reviews which influence readers and thereby affect sales. We consider the problem of identifying spam reviews as a classification problem, i.e., given a review, it needs to be classified either as spam or non-spam.”
The network performed better than currently used spam detection programs with limited labeled data, with accuracy of 71-86 percent when trained on as little as 10 percent of labeled data.
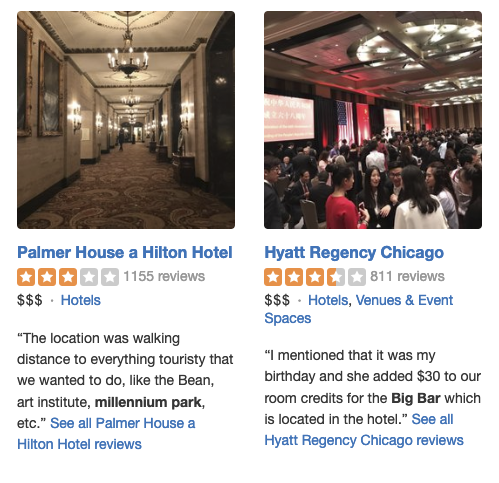
AI can spot fake reviews better than consumers.
Generally speaking, even current AI programs are better than humans at finding fake reviews, according to a story in SciTechEuropa.com. A study conducted by a collaboration between Aalto University’s Secure Systems Research Group and the Waseda University in Japan showed that machine learning models were better than people at finding fake reviews of restaurants on the Yelp app. The university teams presented the paper at the 2018 European Symposium on Research in Computer Security.
“In 2017 researchers at the University of Chicago used a method for training a machine learning model using the data from three million real restaurant ratings on Yelp. After the training, the model was able to generate fake restaurant reviews, but its errors were easily identifiable by readers as it struggled to stay on the right topic, often straying to talk about restaurants in the wrong city. Juuti and his team used neural machine translation instead, to provide the model with context using a text sequence of ‘review rating, restaurant name, city, state, and food tags’.”
A story on Hackernoon.com points out that younger readers, Millennials, are more likely to believe fake news and reviews without examining them for telltale signs. Also, that “90% of the audience knows about fake reviews but only 61% is truly concerned.” Clearly, retailers, restaurants and travel businesses have a stake in making sure that people are informed, making the tools to identify fake reviews of measurable value to them.
Leave A Comment