Olfactory Region May Provide Answer to Expanding AI Capabilities
Today’s artificial intelligence systems, including the artificial neural networks broadly inspired by the neurons and connections of the nervous system, perform wonderfully at tasks with known constraints. They need a lot of computational power and vast quantities of training data to play chess or Go, detect if there’s a car in an image and differentiate between cats and dogs.
“But they are rather pathetic at composing music or writing short stories,” said Konrad Kording, a computational neuroscientist at the University of Pennsylvania. “They have great trouble reasoning meaningfully in the world.”
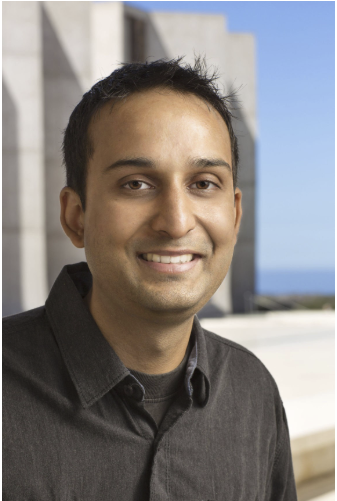
Saket Navlakha, a computer scientist at the Salk Institute, has developed algorithms based on the fly olfactory circuit, in hopes of improving machine learning techniques for similarity searches and novelty detection tasks. Photo: Salk Institute
To overcome those limitations, some research groups are turning to the brain for new ideas. They are choosing what may seem an unlikely starting point: the sense of smell, or olfaction. Scientists trying to gain a better understanding of how organisms process chemical information have uncovered coding strategies that seem especially relevant to problems in AI. Olfactory circuits are similar to more complex brain regions identified as essential to building better machines.
But “every type of stimulus doesn’t get processed in the same way,” said Saket Navlakha, a computer scientist at the Salk Institute for Biological Studies in California. “Vision and olfaction are very different types of signals, for example. … So there may be different strategies to deal with different types of data. I think there could be a lot more lessons beyond studying how the visual system works.”
Olfaction research didn’t take off until the 1990s, when the biologists Linda Buck and Richard Axel, both at Columbia University at the time, discovered the genes for odor receptors. Since then, however, the olfactory system has become particularly well characterized, and it’s something easily studied in flies and other insects. It’s possible to study general computational challenges, some scientists argue.
“Let’s say you have 1,000 people and you stuff them into a room and try to organize them by hobby,” Navlakha said. “Sure, in this crowded space, you might be able to find some way to structure these people into their groups. But now, say you spread them out on a football field. You have all this extra space to play around with and structure your data.”
As researchers studied olfaction in the early 2000s, they developed algorithms to determine how random embedding and sparsity in higher dimensions helped computational efficiency. One pair of scientists, Thomas Nowotny of the University of Sussex in England and Ramón Huerta of the University of California, San Diego, even drew connections to another type of machine learning model, called a support vector machine. They argued that the ways both the natural and artificial systems processed information, using random organization and dimensionality expansion to represent complex data efficiently, were formally equivalent. AI and evolution reached the same solution.
The lengthy Wired magazine article on this remarkable idea and complicated subject was written by Jordana Cepelewitcz.
read more at wired.com
Leave A Comment