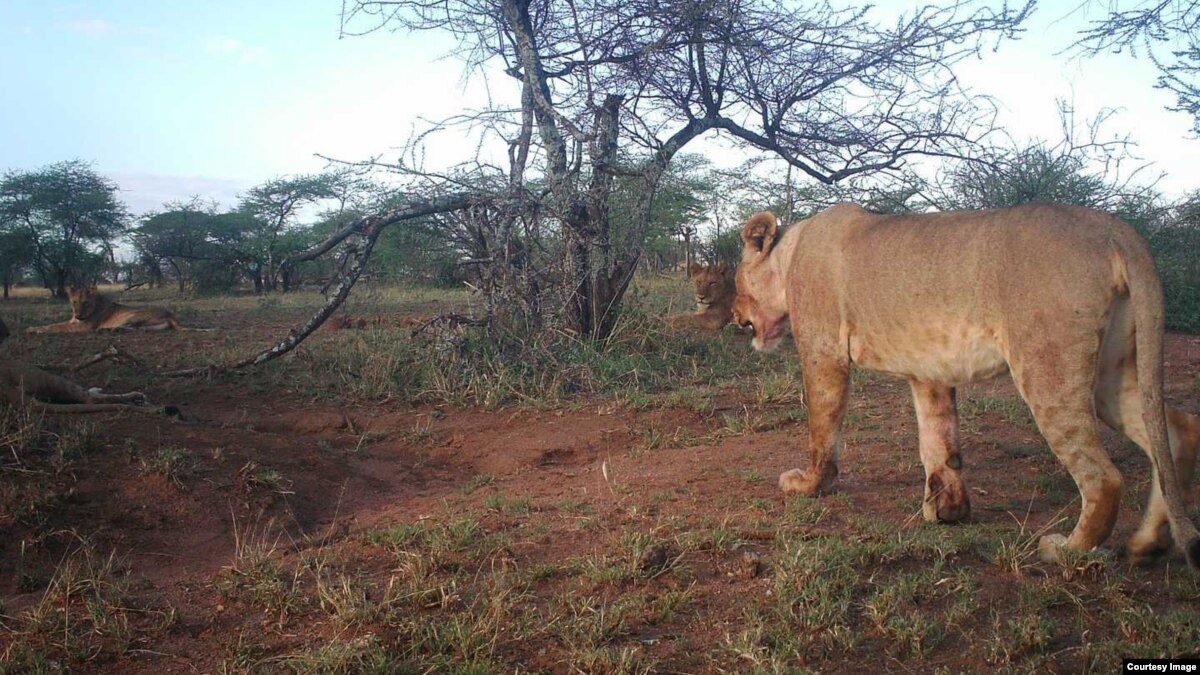
This photo was taken from one of 225 cameras positioned in Tanzania’s Serengeti National Park. One of the largest and longest running camera-trap projects, for more than eight years researchers have operated Snapshot Serengeti. (Snapshot Serengeti)
Tracking Threatened Species with Modern Algorithms
In a recent article in VOA News.com, researchers described how they combined a project for the study of two of the major world problems: climate change and endangered wildlife.
Animal migrations and bird migrations were at first kept track through hand counting as they followed centuries old patterns across Africa and other continents. Then people turned to airplanes, then satellites. Now modern-day algorithms paired with machine learning can identify animals and even track the difference in the way birds sing and chirp.
Scientists have long struggled with how to measure the effects of climate change on wildlife. This is especially true for birds flying in and out of coastal areas bordering the Arctic Ocean.
In the past, researchers depended mainly on information gathered by satellite to follow the movement of birds and animals. But this method can be costly and result in huge amounts of information, which can be difficult to process. Now scientists are turning to another kind of technology to help them follow birds and other wildlife. Songbirds are especially difficult to study in the wild. They can fly across thousands of kilometers and their bodies are too small for electronic tracking devices.
A new report tells about a project in which researchers recorded songbirds and then put the sounds into a computer program, using machine learning algorithms with the ability to focus on bird calls while ignoring other noises. The algorithm helps improve the likelihood of predicting the correct result. Researchers have used similar machine learning methods to recognize human speech.
By tapping into several modern technologies, researchers and scientists have combined some of their projects and are studying results from these collaborations. From machine learning to track song birds, to AI and big data being able to store and analyze this information, the technology gives a far more accurate accounting of herd sizes or the flocks of migrating birds’ movements
The project in Tanzania involves setting cameras and sensors in the areas where animals and birds are known to breed, raise young and all the other movements that migrating herds make.
Oliver says the unsupervised machine learning method could be retooled to use sounds to measure the movements of other wildlife. “This could allow us to track large-scale changes in how animals are responding to climate change,” she said.
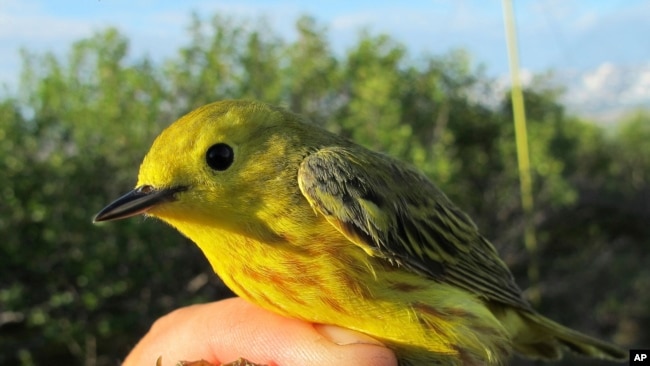
This June 18, 2016 photo provided by the U.S. Geological Survey shows a Yellow Warbler in Nome, Alaska. (Rachel M. Richardson/U.S. Geological Survey via AP)
In another study, researchers reported how machine learning methods can be used to identify, count and describe animals in the wild. Currently, scientists depend mainly on cameras in the wild to keep track of animals. But just like the first study, the second also points out the problems of trying to deal with the large amounts of information gathered. Processing this data can be costly and involve a lot of people. Researchers created a machine learning system they say uses artificial intelligence or AI to identify, count, and describe the behaviors of 48 animal species. They said their method can correctly identify animals at a rate of 94 percent.
By having the luxury of setting up cameras and sensors, and leaving them for long, uninterrupted periods of time, researchers have less chance of spooking the animals they are studying. Using this kind of equipment shows they wont be needing more funding for the support, outfitting, and up-keep of a group of humans, hidden in blinds or chasing after spooked herds. With AI, machine learning and other technologies, scientists are collecting deeper, more accurate data, that can be analyzed and acted upon almost instantly.
Bryan Lynn wrote the original story for Learning English, based on a report from Sadie Witkowski of VOA News and other sources.
read more at learningenglish.voanews.com
GPS Data Alone Accurately Predicts Diving in Seabirds
To further explore research using deep learning, GPS and other technologies to study seabirds and their ecosystems around the world, go to doi.org
Leave A Comment