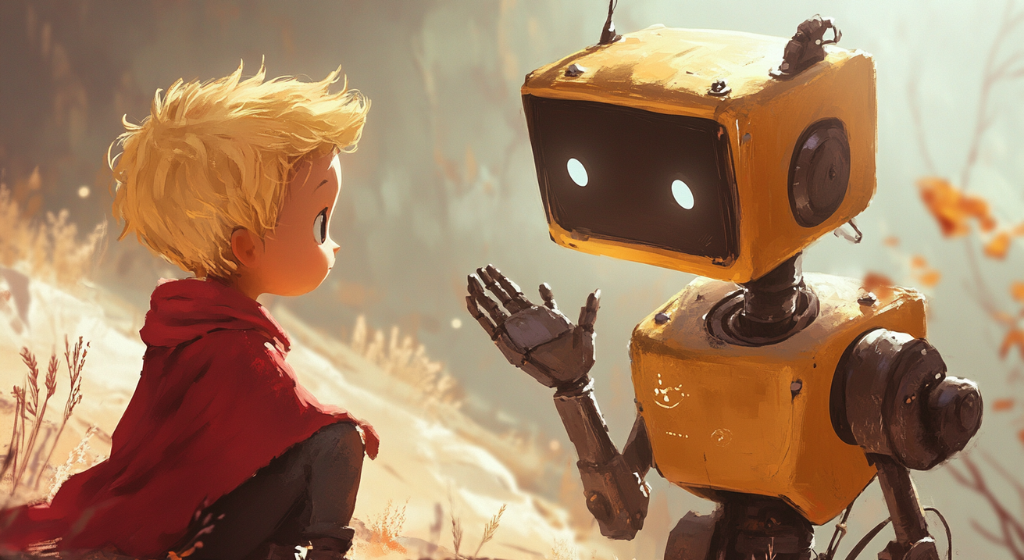
A new study by Meta AI and French researchers finds that AI language models like Llama 3.1 develop linguistic capabilities through stages that closely mirror how children’s brains learn language, shedding light on both machine learning and human cognition. (Source: Image by RR)
Llama 3.1 Shows Neural Parallels to Older Children’s Language Processing
A joint study by Meta AI and several French medical centers has revealed compelling similarities between how children develop language in the brain and how AI models learn language through training. By recording brain activity in 46 French-speaking participants between the ages of 2 and 46 using electrodes implanted for epilepsy treatment, researchers observed how language comprehension develops over time. Participants listened to the audiobook The Little Prince, and neural responses to speech were tracked across more than 7,400 electrodes. The youngest children in the study exhibited early neural reactions to basic speech sounds like “b” and “k,” while more complex understanding—such as word meaning and grammar—was only present in older children and adults, involving more advanced brain regions.
As children aged, language-related neural activity expanded both spatially and temporally across the brain. Responses to language stimuli began occurring sooner, lasted longer, and were more distributed—indicating increasingly sophisticated processing. To understand how these developmental stages relate to machine learning, the team compared the human neural data to responses generated by two AI models: wav2vec 2.0, which learns from raw audio, and Meta’s large language model Llama 3.1. Both AI models, as noted in the-decoder.com, produced low-level responses, but after training, their internal processing began to mirror human brain activity. Llama 3.1, in particular, began resembling the brains of older children and adults, suggesting its ability to grasp whole-word representations post-training.
The research found that AI language models and human brains appear to follow a similar developmental trajectory: beginning with simple sound recognition and progressing toward the comprehension of meaning and structure. For instance, toddlers’ brain activity looked similar to early-stage, untrained AI models, while older children’s and adults’ brains mirrored the architecture and functioning of fully trained language models like Llama 3.1. However, a critical difference remains—human children learn language with dramatically less input, needing only a few million words, whereas AI models require billions of examples to reach comparable performance levels. This underscores the efficiency of biological learning while demonstrating how AI can still serve as a valuable analog for studying cognition.
Despite limitations—such as being unable to include children under age two due to medical constraints—the study opens new avenues for exploring cognitive development. The insights gained could enhance both neuroscience and AI, providing a way to better understand how humans acquire language while refining how machines simulate that process. According to lead researcher Jean-Rémi King, AI models like Llama 3.1 could ultimately help uncover the timeline and structure of language acquisition in humans, offering a unique lens into the evolution of linguistic capability.
read more at the-decoder.com
Leave A Comment