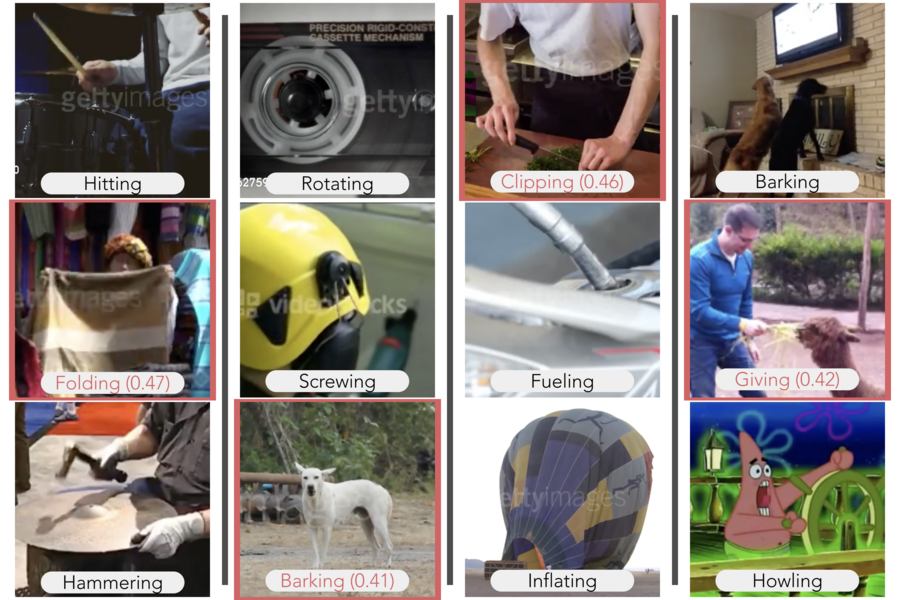
These video images were used to train the ML model on abstract concepts. (Source: MIT CSAIL)
Video Training Lets AI Recognize, Connect Abstract Concepts
While it may not sound like a monumental step forward, the success by MIT’s AI lab in teaching a machine-learning vision model of an algorithm to connect random images and the sounds they make as being conceptually similar is a breakthrough for neural networks. The model also rejected videos that didn’t fit.
To put it into perspective, it’s the closest an AI has come to a human level of intelligence, even though it’s still at the level of a human toddler. As the MIT News explained:
“Organizing the world into abstract categories does not come easily to computers, but in recent years researchers have inched closer by training machine learning models on words and images infused with structural information about the world, and how objects, animals, and actions relate. In a new study at the European Conference on Computer Vision this month, researchers unveiled a hybrid language-vision model that can compare and contrast a set of dynamic events captured on video to tease out the high-level concepts connecting them.”
The ML model did well in identifying video concepts that went together conceptually, such as putting together a set of three videos of a dog barking, a man howling beside his dog and a crying baby out of five videos presented. Researchers at MIT’s Computer Science and Artificial Intelligence Laboratory (CSAIL) trained two AI systems in action recognition: MIT’s Multi-Moments in Time and DeepMind’s Kinetics.
“We show that you can build abstraction into an AI system to perform ordinary visual reasoning tasks close to a human level,” says the study’s senior author Aude Oliva, a senior research scientist at MIT, co-director of the MIT Quest for Intelligence, and MIT director of the MIT-IBM Watson AI Lab. “A model that can recognize abstract events will give more accurate, logical predictions and be more useful for decision-making.”
A story on edgy.app explained another concept the model put together from WordNet, a database of word meanings, to map each action-class label’s relation in their dataset.
“For example, they linked words like ‘sculpting,’ ‘carving,’ and ‘cutting’ to higher-level concepts such as ‘crafting,’ ‘cooking,’ and ‘making art.’ So, when the model recognizes sculpting activity, it can pick out conceptually similar activities.”
These basic abilities are bringing AI closer to the human ability to compare and to contrast, according to the study’s senior author Aude Oliva, a senior research scientist at MIT, co-director of the MIT Quest for Intelligence and MIT director of the MIT-IBM Watson AI Lab.
“It’s a rich and efficient way to learn that could eventually lead to machine learning models that can understand analogies and are that much closer to communicating intelligently with us.”
read more at news.mit.edu
Leave A Comment