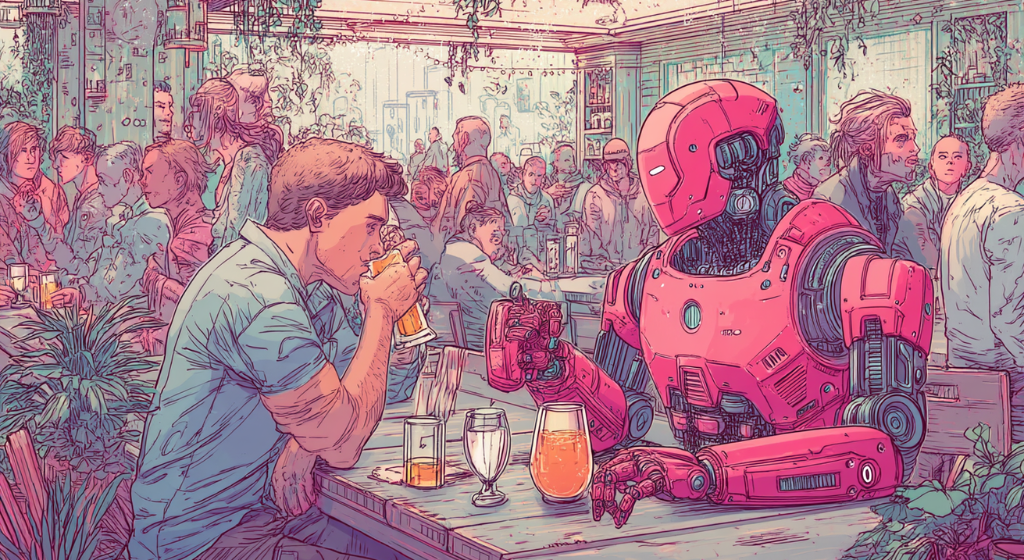
A Johns Hopkins study reveals that AI models, despite their rapid advances, still dramatically lag behind humans in interpreting dynamic social interactions, highlighting a fundamental shortcoming for future AI-human collaboration. (Source: Image by RR)
Current Neural Networks May Be Fundamentally Flawed for Dynamic Environments
Researchers at Johns Hopkins University have found that humans outperform current AI models when it comes to interpreting social interactions in dynamic, real-world scenes—a crucial skill needed for technologies like self-driving cars, assistive robots and other human-facing AI systems. Led by cognitive science professor Leyla Isik, the study highlights how AI struggles to recognize human intentions, goals, and behaviors, such as determining if a pedestrian is about to cross the street or if two individuals are simply engaged in conversation. The findings, as noted in techxplore.com, suggest a fundamental gap in AI’s ability to comprehend social dynamics, raising concerns about deploying AI in environments that require nuanced human interaction.
The study, presented at the International Conference on Learning Representations and published in PsyArXiv, involved human participants watching short video clips and rating social features like interaction and intention. Researchers compared these human assessments with predictions made by over 350 AI models, spanning language, image, and video analysis. Across the board, humans consistently agreed with each other’s interpretations, while AI models, regardless of their size or training data, failed to accurately predict human judgments. Notably, video-based AI models performed poorly at describing activities, and even when provided a series of still frames, image models could not reliably detect human communication.
While large language models showed some relative strength in predicting human behavior, and video models were slightly better at predicting patterns of neural activity in the brain, none of the AI systems came close to matching human consistency across all tasks. This contrasts sharply with AI’s previous successes in interpreting static images, revealing a significant shortcoming in dynamic scene understanding. Researchers emphasized that real-life interactions are fluid and involve complex, evolving contexts that today’s AI systems are poorly equipped to comprehend.
The root of the problem may lie in the very architecture of AI neural networks, which were primarily modeled after parts of the brain responsible for processing static images, not dynamic, social scenarios. “There’s something fundamental about the way humans process scenes that these models are missing,” Isik concluded. The research suggests that for AI to truly integrate into human environments, it must evolve beyond static object recognition toward deeper, narrative-based social understanding—a challenge that demands rethinking how AI systems are designed.
read more at techxplore.com
Leave A Comment