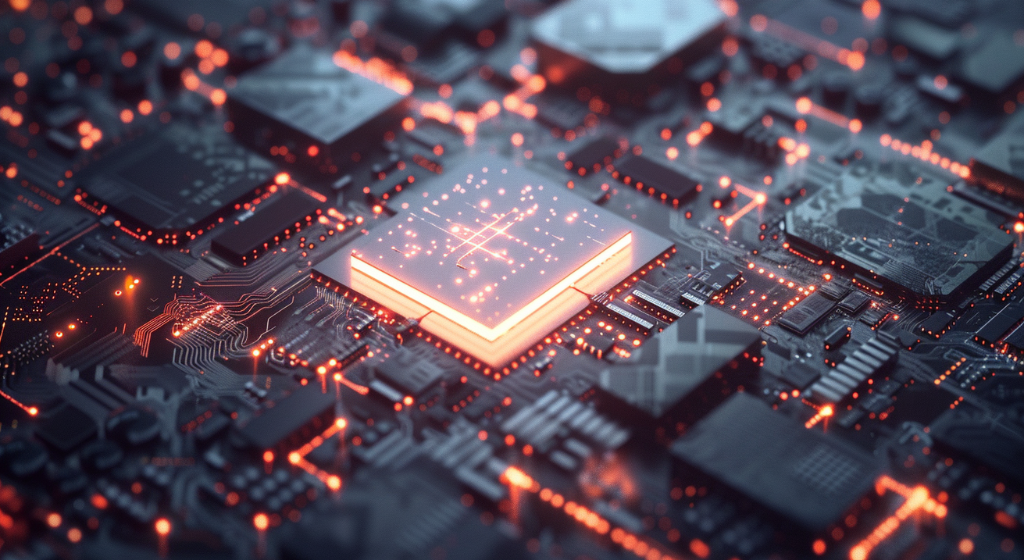
Although HITOP is far from matching the performance of electronic predecessors, with HITOP performing about 1 trillion operations per second compared to Nvidia chips handling 300 times more data, its compelling efficiency lies in reducing energy costs by 1,000 times. (Source: Image by RR)
Pioneering Research in Photonics Brings New Hope for Sustainable AI Development
Researchers are exploring optical neural networks (ONNs) to address the increasing energy demands of AI systems. Unlike traditional electronic chips, which use electrons, ONNs use photons for processing, potentially offering significant efficiency gains. This shift, as reported in wired.com, is driven by the limitations of Moore’s law and the growing computational needs of AI, which are predicted to consume substantially more power in the coming years.
Optical computing, particularly matrix multiplication with photons, shows promise for high-speed, energy-efficient processing. Initial experiments have demonstrated the potential for light-based systems to outperform electronic devices in specific tasks, such as recognizing spoken vowels. However, significant challenges remain, including the difficulty of scaling these systems and managing the energy costs associated with converting electronic signals to optical ones. The 2017 paper from MIT showcased an ONN that encoded quantities into beams of light for matrix multiplication, sparking renewed interest and advancements in the field.
Recent advancements, like the HITOP system from MIT, aim to enhance the throughput of optical networks by using three-dimensional light data, reducing energy costs per calculation. This system can run machine-learning models 25,000 times larger than previous optical neural networks by packing more data into the light signals. Additionally, other groups have developed flexible optical computers that can be reprogrammed on the fly using lasers, allowing for dynamic changes in the computation process. These innovations highlight the potential of ONNs to handle complex tasks more efficiently than electronic systems, although they currently fall short in processing power compared to advanced electronic chips from companies like Nvidia.
The future of ONNs may lie in specialized applications, such as counteracting wireless transmission interference. For example, an ONN developed by researchers at Queen’s University can sort out different wireless signals with a processing delay of under 15 picoseconds, significantly faster and more energy-efficient than electronic systems. Researchers remain optimistic about the potential for ONNs to eventually surpass electronic systems in efficiency and performance, envisioning significant gains within the next decade. If successful, ONNs could make AI models more than 1,000 times as efficient as future electronic systems, representing a major breakthrough in the field of computing.
read more at wired.com
Leave A Comment