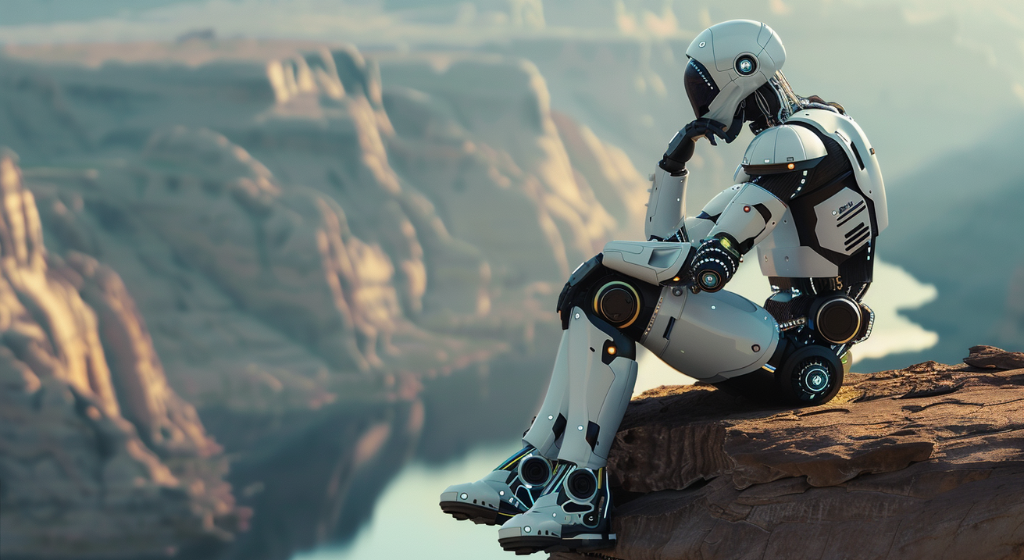
OpenAI has developed a new method to break down GPT-4’s inner workings into 16 million human-understandable patterns, enhancing the understanding of AI models’ safety and robustness, although large AI models remain largely opaque in their functionality. (Source: Image by RR)
Advanced AI Analysis: OpenAI and Anthropic’s Race to Understand Neural Networks
OpenAI has developed a new method to break down the inner workings of GPT-4 into 16 million patterns, making it easier for humans to understand its functionality. Despite significant advancements, large AI models like GPT-4 remain “black boxes,” functioning without clear insights into their internal processes. OpenAI’s new approach involves using “sparse autoencoders,” neural networks that learn to reconstruct input data, to identify patterns of activity in GPT-4’s neural networks that can be interpreted by humans.
The autoencoder learns to translate complex activation patterns into more compact, interpretable features by filtering out the most important ones. This method allows researchers to match each learned feature to a human-understandable concept, such as grammar rules, world facts, or logical reasoning, thus providing insights into how GPT-4 “thinks.” The primary challenge, as noted in the-decoder.com, was scaling the autoencoders to handle millions of features, which OpenAI has managed to achieve with a 16 million feature autoencoder.
OpenAI found specific features in GPT-4 related to human flaws, price increases, ML training logs, and algebraic rings, though many features were difficult to understand or showed unrelated activity. Despite this progress, the sparse autoencoder doesn’t capture all the original model’s capabilities. Scaling to billions or trillions of features would be necessary to fully understand GPT-4’s inner workings, a task that remains challenging even with improved scaling techniques.
OpenAI has published its findings, released the source code on GitHub, and built an interactive visualizer for the learned features. Competitor Anthropic has conducted similar research, highlighting the importance of interpretability for both safety and performance in AI models. However, scaling these analysis methods remains a significant hurdle, requiring far more computing power than training the models themselves.
read more at the-decoder.com
Leave A Comment