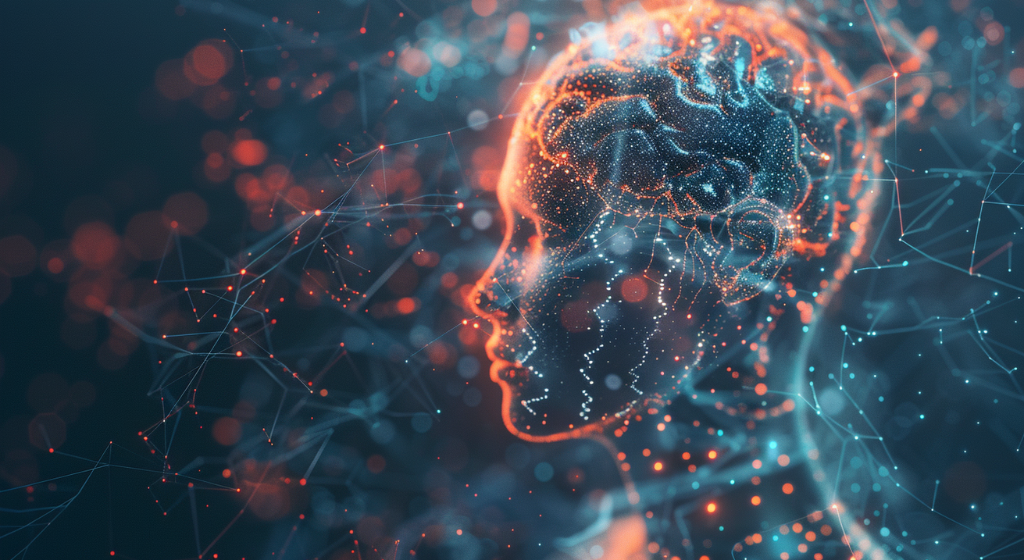
The ubiquity of generative AI, like ChatGPT, Gemini, and Anthropic’s Claude, which impresses with language skills but can generate misinformation and dangerous content, underscores the need for understanding these “black boxes” to improve safety and prevent harmful outputs. (Source: Image by RR)
Anthropic’s Research Marks a Crucial Step Towards Greater Transparency in AI
Anthropic, an AI startup co-founded by Chris Olah, has made significant progress in understanding the internal workings of artificial neural networks, which have long been considered black boxes. Olah, who has been fascinated by neural networks throughout his career, leads a team that has managed to reverse engineer large language models (LLMs) to identify specific outputs. Their research has pinpointed combinations of artificial neurons that correspond to various concepts, such as burritos, semicolons in programming code, and even dangerous topics like biological weapons, which could potentially enhance AI safety by identifying and mitigating risks within these models.
The team’s approach involves treating artificial neurons like letters that form meaningful words when combined, using a technique called dictionary learning to associate neuron combinations with specific concepts, or “features.” According to a story in wired.com, this method allowed them to decode a simplified model before tackling a full-sized LLM, Claude Sonnet, identifying millions of features, including safety-related ones. They found that manipulating these neural features could alter the model’s behavior, making it possible to enhance safety and reduce biases by suppressing harmful features, though turning up certain features too much could lead to extreme and undesirable outputs.
Anthropic’s work is part of a broader effort within the AI research community to make neural networks more transparent and understandable. Other teams, such as those at DeepMind and Northeastern University, are also working on similar projects, employing different techniques to crack open the black box of LLMs. These efforts collectively aim to provide better control over AI systems, ensuring they are safer and more reliable, though there remain significant challenges and limitations in fully decoding these complex models.
While Anthropic’s research represents a promising step forward, the team acknowledges that their work is far from complete, and there are inherent limitations in their approach. The techniques used may not be universally applicable to all LLMs, and identifying all possible features remains a challenge. Despite these hurdles, the progress made by Anthropic and similar research initiatives marks a crucial advancement in the quest to understand and safely manage the inner workings of AI systems, shedding light on what has been a largely mysterious field.
read more at wired.com
Leave A Comment