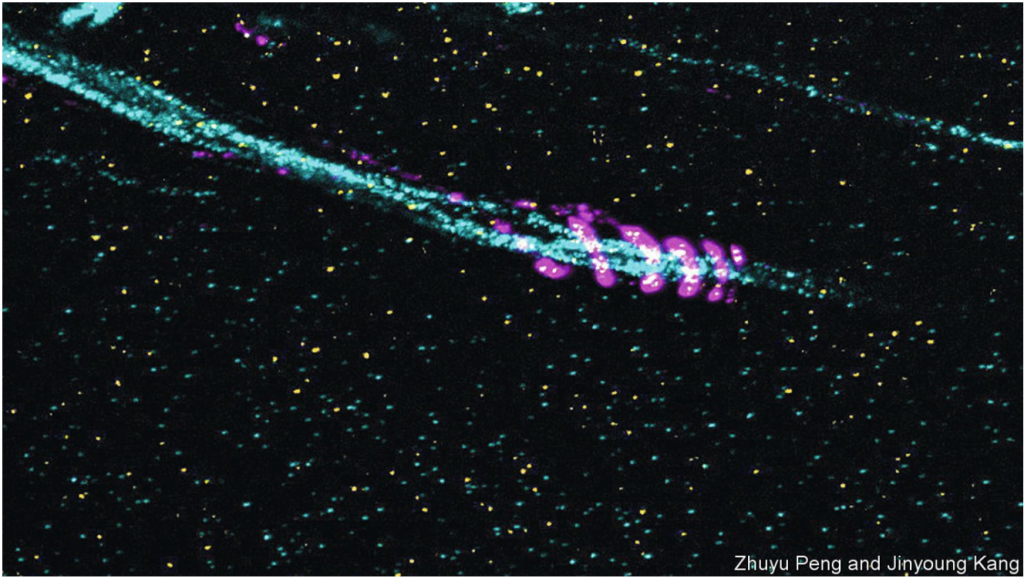
A microscropic image of a protein provided by researchers for economist.com, found through machine learning. (Source: Zhuyu Peng, Jinyoung Kang)
AlphaFold Found the Proteins, but Other Programs May Improve on Creating New Drugs
While DeepMind’s AlphaFold model has predicted nearly all known protein structures discovered yet, it’s not a tool that can help scientists invent drugs yet, partly due to its limitations in modeling how proteins and drugs would interact, according to an analysis by MIT researchers, reported on theregister.com.
That’s not to diminish what AlphaFold has achieved, which the scientists make clear. The program has now predicted over 200 million proteins from their amino acid strings.
“Breakthroughs such as AlphaFold are expanding the possibilities for in silico (computer simulation) drug discovery efforts, but these developments need to be coupled with additional advances in other aspects of modeling that are part of drug discovery efforts,” James Collins, lead author of the study published in Molecular Systems Biology and a bioengineering professor at MIT, said in a statement. “Our study speaks to both the current abilities and the current limitations of computational platforms for drug discovery.”
However, researchers have a long way to go to find the best way to leverage those discoveries with physical reality.
“Breakthroughs such as AlphaFold are expanding the possibilities for in silico (computer simulation) drug discovery efforts, but these developments need to be coupled with additional advances in other aspects of modeling that are part of drug discovery efforts,” James Collins, lead author of the study published in Molecular Systems Biology and a bioengineering professor at MIT, said in a statement.
“Our study speaks to both the current abilities and the current limitations of computational platforms for drug discovery.”
The initial story on AlphaFold’s discoveries and what they mean, published on MIT’s technologyreview.com make its importance clear, but since then a new program has been developed that could further the efforts to find new drugs to treat diseases. A story on economist.com explores a new microscope that makes tiny protein structures visible, which could help advance research beyond AlphaFold’s findings. Also, other machine learning models could surpass those of AlphaFold, according to Felix Wong, co-author of the paper and a postdoctoral researcher at MIT.
“The machine-learning models learn not just the shapes, but also chemical and physical properties of the known interactions, and then use that information to reassess the docking predictions,” Wong said. “We found that if you were to filter the interactions using those additional models, you can get a higher ratio of true positives to false positives.”
read more at the register.com
Leave A Comment